A MRI brain tumor image segmentation method based on optimized U-net network model
A network model and image segmentation technology, applied in the field of brain tumor image segmentation, can solve the problems of medical diagnosis and treatment, increase the difficulty of signal-to-noise distinction, and reduce the accuracy of segmentation, and achieve fast segmentation, low cost, and improved accuracy. Effect
- Summary
- Abstract
- Description
- Claims
- Application Information
AI Technical Summary
Problems solved by technology
Method used
Image
Examples
Embodiment 1
[0075] like Figure 5 Described, the present embodiment discloses a kind of MRI brain tumor image segmentation method based on optimized U-net network model, and described method comprises:
[0076] 101. Preprocessing the acquired multimodal MRI brain tumor image data.
[0077] The preprocessing process here is to remove the noise signal of the multimodal MRI brain tumor image data, and make it more closely match the algorithm of the model, so as to achieve the purpose of improving the quality of the final output image.
[0078] 102. Input the preprocessed multimodal MRI brain tumor image data into the trained U-net network model.
[0079] It should be noted that the U-net network model described here is an improved U-net network model, and it is a U-net network model after a large number of brain tumor images and cell structure images are trained.
[0080] 103. Obtain the multimodal MRI brain tumor image segmentation data output by the U-net network model.
[0081] Here, t...
Embodiment 2
[0112] This embodiment combines the deep convolutional neural network and migration learning to improve the U-Net network model, and then uses the data set generated after simple data amplification, normalization and other preprocessing of the cell structure image to improve the U-Net network model. net network model for pre-training, and then use the multi-modal MRI brain tumor images (including HGG220 sets and LGG 54 sets) used in the BRATS2015 competition to fine-tune the network after preprocessing, and finally generate a multi-modal A deep learning model for segmentation of MRI brain tumor images. Framework flow chart such as Figure 8 shown.
[0113] Transfer learning: Deep learning has achieved major breakthroughs in computer vision tasks due to the use of very large datasets such as ImageNet to train networks. To effectively utilize smaller datasets, it is common to train a base model on a large dataset (such as ImageNet) and then fine-tune the learned model on a sec...
PUM
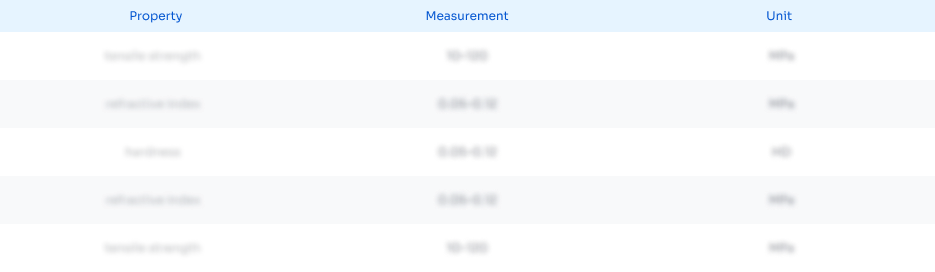
Abstract
Description
Claims
Application Information
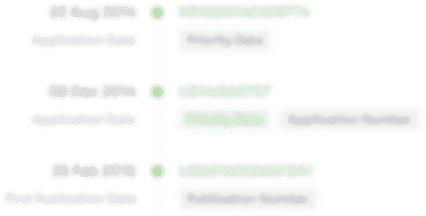
- Generate Ideas
- Intellectual Property
- Life Sciences
- Materials
- Tech Scout
- Unparalleled Data Quality
- Higher Quality Content
- 60% Fewer Hallucinations
Browse by: Latest US Patents, China's latest patents, Technical Efficacy Thesaurus, Application Domain, Technology Topic, Popular Technical Reports.
© 2025 PatSnap. All rights reserved.Legal|Privacy policy|Modern Slavery Act Transparency Statement|Sitemap|About US| Contact US: help@patsnap.com