Multi-task learning cell counting method based on convolutional neural network
A convolutional neural network and multi-task learning technology, applied in the field of multi-task learning cell counting, can solve problems such as low efficiency, achieve strong robustness, improve efficiency, and improve performance
- Summary
- Abstract
- Description
- Claims
- Application Information
AI Technical Summary
Problems solved by technology
Method used
Image
Examples
Embodiment Construction
[0037] The main idea of the present invention is to fully consider the relationship between the overall features and local features of the cell image, embody the superiority of the feature fusion of the corresponding layers between multi-column convolutions, further enhance the robustness of cell counting, and at the same time improve the cell count through multi-task learning. Counting accuracy.
[0038] Such as figure 1 As shown, the present invention provides a kind of multi-task learning cell counting method based on convolutional neural network, and it comprises following four steps:
[0039] Step S1: Preprocessing the biological cell image data set;
[0040] Specifically, we first obtain the pictures of biological cells under the microscope. The size of the pictures can be any size, and they can be used as input pictures. The annotation of each cell picture includes two parts, which are the cell coordinate points in each picture and the actual total number of cells. ...
PUM
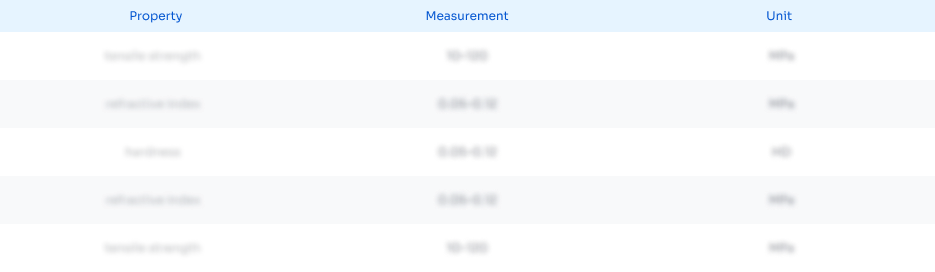
Abstract
Description
Claims
Application Information
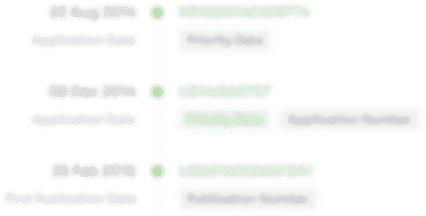
- R&D Engineer
- R&D Manager
- IP Professional
- Industry Leading Data Capabilities
- Powerful AI technology
- Patent DNA Extraction
Browse by: Latest US Patents, China's latest patents, Technical Efficacy Thesaurus, Application Domain, Technology Topic, Popular Technical Reports.
© 2024 PatSnap. All rights reserved.Legal|Privacy policy|Modern Slavery Act Transparency Statement|Sitemap|About US| Contact US: help@patsnap.com