Hyperspectral band selection method based on separable convolution and hard threshold function
A band selection and hard threshold technology, applied in image processing and agriculture, can solve the problems of slow speed, poor band selection performance, low classification accuracy, etc., to improve classification accuracy, speed up band selection and classification speed, and fast classification speed. Effect
- Summary
- Abstract
- Description
- Claims
- Application Information
AI Technical Summary
Problems solved by technology
Method used
Image
Examples
Embodiment 1
[0036] Because hyperspectral data records continuous spectral information and has high spectral resolution, it has a wide range of applications in military surveys, ecological construction, land use, global environment, natural disasters, and other fields. However, due to the high resolution of the hyperspectral image spectrum, it has a high spectral dimension and a large amount of data, which brings many problems to the processing of hyperspectral images, such as increasing information redundancy and data storage. space, and prolong the data processing time. In addition, hyperspectral images contain noise or invalid bands caused by interference from other factors during the radiation process. Crucially, when the number of image samples is small and the amount of processed data is large, It is easy to produce the phenomenon of "curse of dimensionality". Therefore, it is extremely necessary to reduce the number of bands in hyperspectral images.
[0037] There are existing hype...
Embodiment 2
[0059] The hyperspectral band selection method based on separable convolution and hard threshold function is the same as embodiment 1, and the construction band selection layer described in step (4a) is used as the first layer of the convolutional neural network based on band selection, including the following steps :
[0060] (4a1) The convolutional neural network based on band selection has a total of ten layers. Among them, the band selection layer based on separable convolution is the first layer, and the space-spectrum joint information extraction layer based on multi-scale convolution is the second layer. Based on three A classifier with one convolutional layer, two pooling layers, two fully connected layers and one softmax classification layer is used as the follow-up network.
[0061] The number of network layers above can also be modified, but it is worth noting that if the number of network layers is too small, the nonlinear classification ability of the network will...
Embodiment 3
[0066] The hyperspectral band selection method based on separable convolution and hard threshold function is the same as embodiment 1-2, and the band selection layer described in step (4b) utilizes separable convolution and hard threshold function to select bands, including the following steps:
[0067] (4b1) Arrange the weight matrix of the band selection layer in descending order to obtain a one-dimensional vector S with a size of 1×200, determine the number N of selected bands, and use the elements at the numerical positions of the bands in the sorted vector as thresholds, That is, the element at the Nth position in the vector S is taken, and a hard threshold function based on the threshold is established.
[0068] (4b2) Enter the hard threshold function obtained by inputting each element in the weight matrix of the band selection layer. Specifically, for the weight value greater than or equal to the threshold value, the output retains the original value, otherwise it is set...
PUM
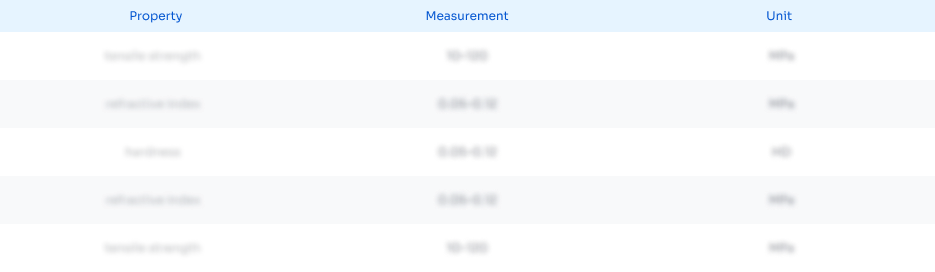
Abstract
Description
Claims
Application Information
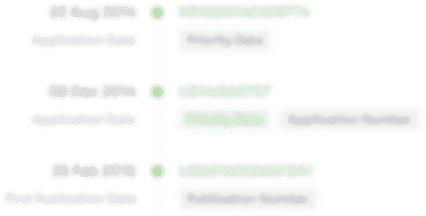
- R&D Engineer
- R&D Manager
- IP Professional
- Industry Leading Data Capabilities
- Powerful AI technology
- Patent DNA Extraction
Browse by: Latest US Patents, China's latest patents, Technical Efficacy Thesaurus, Application Domain, Technology Topic, Popular Technical Reports.
© 2024 PatSnap. All rights reserved.Legal|Privacy policy|Modern Slavery Act Transparency Statement|Sitemap|About US| Contact US: help@patsnap.com