Functional Magnetic Resonance Clustering Imaging Method Based on Gaussian Hidden Variable Dimensionality Reduction Clustering Center
A functional magnetic resonance and clustering center technology, applied in the field of medical imaging, can solve the problems of insufficient memory and slow operation, and achieve the effect of reducing the amount of processed data, avoiding memory overflow, and reducing the occupation of computing resources.
- Summary
- Abstract
- Description
- Claims
- Application Information
AI Technical Summary
Problems solved by technology
Method used
Image
Examples
Embodiment 1
[0045] Example 1: see figure 1 , a functional magnetic resonance clustering imaging method based on Gaussian latent variable dimensionality reduction clustering centers, comprising the following steps:
[0046] (1) collect functional magnetic resonance data of the brain, and preprocess the functional image data of the functional magnetic resonance of the brain, and obtain the preprocessed data set X, Among them, I is the number of voxels in the data set X, x i is the time series of the i-th voxel, the time series length of each voxel is N, I>>N;
[0047] The preprocessing is as follows: the functional imaging data of brain functional magnetic resonance are firstly corrected for head movement, normalized to the EPI template, spatially smoothed, and then the low-frequency noise of the signal is filtered out;
[0048] (2) Estimate the essential dimension of the principal component eigenvalues of the covariance matrix of the Z score of the data set X, determine the optimal di...
Embodiment 2
[0069] (1) Sampling to obtain the functional magnetic resonance data of the brain, the functional items of the functional magnetic resonance data of the brain are first corrected for head movement, normalized to the EPI template, smoothed in space, and then the low-frequency noise of the signal is filtered out to obtain the preprocessed data set X , at this time, the data set X is a matrix with I rows and N columns;
[0070] (2) Then proceed to the following steps:
[0071] (21) X is normalized to Z score, and calculates covariance matrix C to Z score matrix, at this moment, covariance matrix C is N rows and N columns, and data volume ratio matrix X (1 row N columns) reduces greatly;
[0072] (22) Estimate the essential dimension of the principal component eigenvalues of the covariance matrix C, determine the optimal dimension M, use the optimal dimension to perform Gaussian process hidden variable analysis and dimension reduction on the covariance matrix, and obtain the dim...
PUM
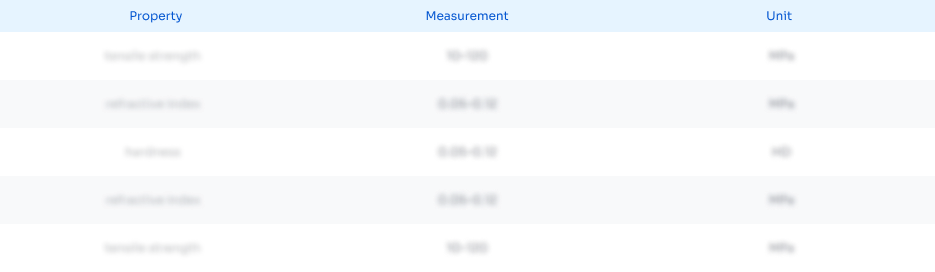
Abstract
Description
Claims
Application Information
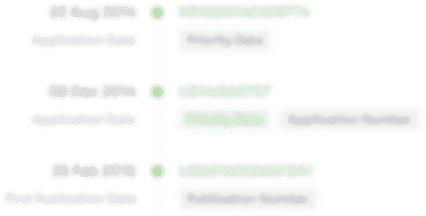
- R&D
- Intellectual Property
- Life Sciences
- Materials
- Tech Scout
- Unparalleled Data Quality
- Higher Quality Content
- 60% Fewer Hallucinations
Browse by: Latest US Patents, China's latest patents, Technical Efficacy Thesaurus, Application Domain, Technology Topic, Popular Technical Reports.
© 2025 PatSnap. All rights reserved.Legal|Privacy policy|Modern Slavery Act Transparency Statement|Sitemap|About US| Contact US: help@patsnap.com