An image conversion method and system based on a generative adversarial network and a ResNts technology
An image conversion and generative technology, applied in the field of image processing, can solve the problems of inaccurate estimation of discriminator density ratio, failure to find the correlation between source domain and target domain features, and unstable training, so as to reduce image artifacts and enhance Stability, high stability effect
- Summary
- Abstract
- Description
- Claims
- Application Information
AI Technical Summary
Problems solved by technology
Method used
Image
Examples
Embodiment Construction
[0062] In order to make the object, technical solution and advantages of the present invention more clear, the present invention will be further described in detail below in conjunction with the examples. It should be understood that the specific embodiments described here are only used to explain the present invention, not to limit the present invention.
[0063] The traditional ResNets structure is difficult to extract the feature information of the low-dimensional space of the input image, which makes the image conversion based on this structure difficult to generate high-resolution images, and the generated images lack details and realistic textures. In addition, the training speed of the network is slow.
[0064] Traditional image conversion algorithms based on GAN, DCGAN, BlurGAN, etc. often can only achieve a single conversion style, and cannot achieve multiple image conversion tasks.
[0065] In view of the above problems, the application of the present invention will...
PUM
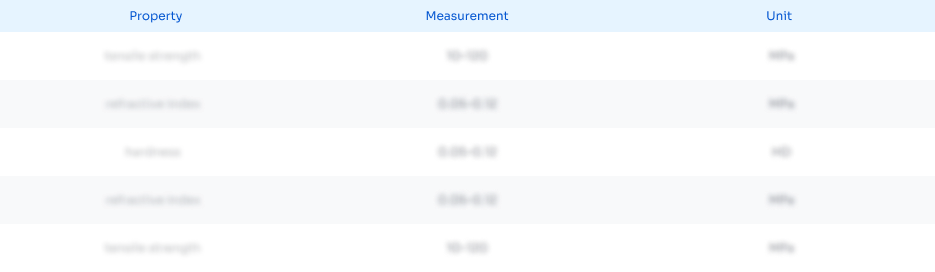
Abstract
Description
Claims
Application Information
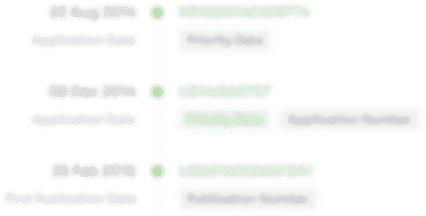
- R&D Engineer
- R&D Manager
- IP Professional
- Industry Leading Data Capabilities
- Powerful AI technology
- Patent DNA Extraction
Browse by: Latest US Patents, China's latest patents, Technical Efficacy Thesaurus, Application Domain, Technology Topic, Popular Technical Reports.
© 2024 PatSnap. All rights reserved.Legal|Privacy policy|Modern Slavery Act Transparency Statement|Sitemap|About US| Contact US: help@patsnap.com