Key evidence extraction method for text prediction result
A text prediction and key technology, applied in the field of key evidence extraction of text prediction results, can solve problems such as wasting time and resources
- Summary
- Abstract
- Description
- Claims
- Application Information
AI Technical Summary
Problems solved by technology
Method used
Image
Examples
specific Embodiment approach 1
[0041] Specific implementation mode one: combine figure 1 Describe this implementation mode, the specific implementation mode is a key evidence extraction method of a text prediction result (see details Figure 4 ), the specific process is:
[0042] Step 1. Find the word vector corresponding to each word from the GloVe word vector (pre-trained vector matrix) for each word, and then encode the sentence through a convolutional neural network to obtain a sentence-level vector;
[0043] Step 2, the sentence-level vector obtained in step 1 averaged to get a sentence-level vector The average of the sentence-level vector The average value of is used as the initial value of the external storage unit;
[0044] Use external storage units to record and accumulate information to support final predictions;
[0045] Inspired by the success of memory networks in the field of question answering, an external memory block is proposed to record information. The external memory block ca...
specific Embodiment approach 2
[0052] Specific embodiment two: the difference between this embodiment and specific embodiment one is that in the step one, the word vector corresponding to each word is searched for each word from the GloVe word vector (pre-trained vector matrix), and then through the volume The product neural network is used to encode sentences to obtain sentence-level vectors; the specific process is:
[0053] Find the word vector corresponding to each word from the pre-trained vector matrix, and then encode the sentence through the convolutional neural network to obtain the vector representation of the sentence; the sentence encoder is not task-specific, but can be semantically combined. Any algorithm for dense vector representation. To improve efficiency, a convolutional neural network is adopted, which is outstanding in various sentence classification tasks, such as sentence-level sentiment analysis. Empirical studies show that convolutional filters with different window widths can capt...
specific Embodiment approach 3
[0062] Embodiment 3: The difference between this embodiment and Embodiment 1 or 2 is that in Step 3, the first sentence-level vector obtained in Step 1, the initial external storage unit obtained in Step 2, and the hard extraction network model are obtained. The updated external storage unit corresponding to the first sentence-level vector;
[0063] Based on the second sentence-level vector obtained in step 1, the updated external storage unit, and the hard extraction network model, the updated external storage unit corresponding to the second sentence-level vector is obtained;
[0064] Until the final external storage unit corresponding to the nth sentence-level vector is obtained based on the nth sentence-level vector obtained in step 1, the updated external storage unit, and the hard extraction network model, the document-level vector is obtained; the specific process is:
[0065] Hard Extraction Network Hard Extra-iNet: The sentence extraction representation module in Hard...
PUM
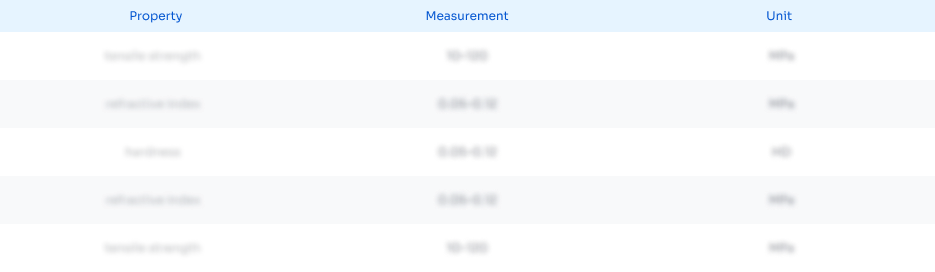
Abstract
Description
Claims
Application Information
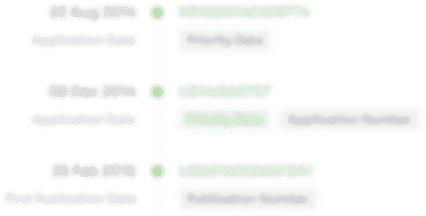
- R&D Engineer
- R&D Manager
- IP Professional
- Industry Leading Data Capabilities
- Powerful AI technology
- Patent DNA Extraction
Browse by: Latest US Patents, China's latest patents, Technical Efficacy Thesaurus, Application Domain, Technology Topic, Popular Technical Reports.
© 2024 PatSnap. All rights reserved.Legal|Privacy policy|Modern Slavery Act Transparency Statement|Sitemap|About US| Contact US: help@patsnap.com