Unmanned aerial vehicle target recognition and classification method based on deep learning
A technology of target recognition and deep learning, which is applied in the field of UAV radio signal monitoring, can solve the problems of unstable target recognition and low signal-to-noise ratio, increase the monitoring range and detection efficiency, improve anti-noise performance, and improve The effect of target recognition accuracy
- Summary
- Abstract
- Description
- Claims
- Application Information
AI Technical Summary
Problems solved by technology
Method used
Image
Examples
Embodiment 1
[0031] Such as figure 1 As shown, the overall workflow of UAV signal recognition classification is as follows:
[0032] After the radio signal sent by the drone is detected by the scout, the data is collected and sent to the data processing center. After signal preprocessing and deep learning algorithm analysis, the target recognition and classification results of the drone are obtained, and the analysis results are combined with the collected data. The data is stored together in the database.
[0033] Such as figure 2 As shown, a deep learning algorithm architecture diagram for UAV target recognition and classification is given. The deep neural network algorithm consists of an input layer, three hidden layers, and an output layer. The detected UAV signal Perform preprocessing such as resampling and Fourier transform to obtain time-domain waveform (data length is n) and spectral feature data (data length is N) respectively. n+N neurons. The time and frequency domain data ...
Embodiment 2
[0036] Such as image 3 As shown, this embodiment provides another deep learning algorithm architecture diagram for UAV target recognition and classification. The deep neural network algorithm is composed of an input layer, a stacked autoencoder, a fully connected layer, and an output layer. The stacked autoencoder consists of a two-layer autoencoder network. The detected UAV signal is subjected to preprocessing such as resampling and Fourier transform to obtain time-domain waveform (data length is n) and spectral characteristic data (data length is N). The time domain and spectrum data pass through two layers of stacked autoencoders respectively, and after information mining in the time domain or frequency domain, they are merged, input to the fully connected layer, and finally to the output layer. The output layer is the probability of each target category, and the category with the highest selection probability is the target recognition result of the UAV signal. Among the...
PUM
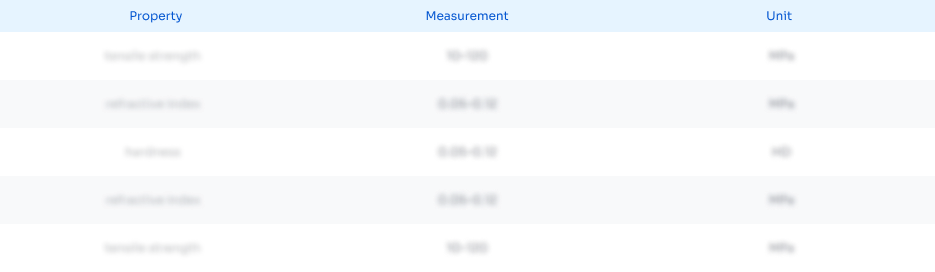
Abstract
Description
Claims
Application Information
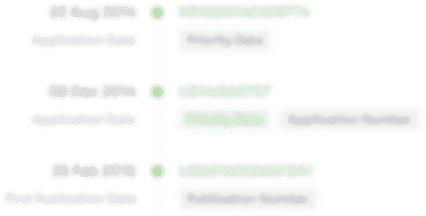
- R&D Engineer
- R&D Manager
- IP Professional
- Industry Leading Data Capabilities
- Powerful AI technology
- Patent DNA Extraction
Browse by: Latest US Patents, China's latest patents, Technical Efficacy Thesaurus, Application Domain, Technology Topic, Popular Technical Reports.
© 2024 PatSnap. All rights reserved.Legal|Privacy policy|Modern Slavery Act Transparency Statement|Sitemap|About US| Contact US: help@patsnap.com