Automobile sensor fault detection method based on independent component analysis and sparse denoising auto-encoder
An independent component analysis, automotive sensor technology, applied in neural learning methods, instruments, special data processing applications, etc., to achieve the effect of enhancing robustness, full utilization, and improving accuracy
- Summary
- Abstract
- Description
- Claims
- Application Information
AI Technical Summary
Problems solved by technology
Method used
Image
Examples
Embodiment Construction
[0020] The present invention will be further described below in conjunction with accompanying drawing.
[0021] The present invention is aimed at the fault detection problem of automobile process, at first utilizes vehicle data collection system to collect the data under the normal working condition as training data set, then utilizes FastICA algorithm to extract the non-Gaussian information in data set to calculate I 2 statistic and get the statistical limit of detection Using sparse denoising autoencoder to extract Gaussian information in residual space to calculate H 2 statistic and get the statistical limit of detection Store all process model parameters in the database for future use. When detecting new online process data, FastICA and sparse denoising self-encoder are also used to obtain corresponding detection results.
[0022] The main steps of the automobile sensor fault detection method based on independent component analysis and sparse denoising self-encoder of...
PUM
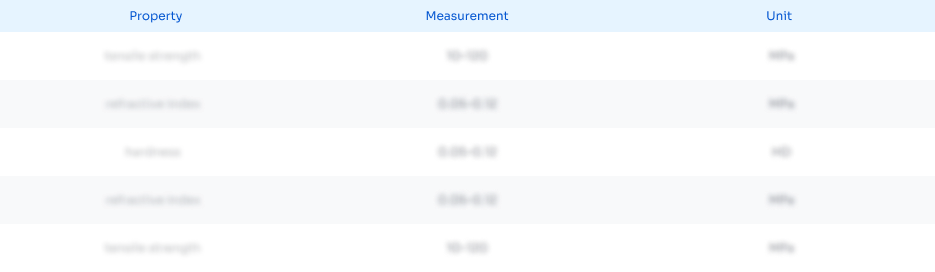
Abstract
Description
Claims
Application Information
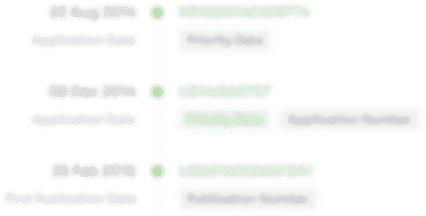
- R&D Engineer
- R&D Manager
- IP Professional
- Industry Leading Data Capabilities
- Powerful AI technology
- Patent DNA Extraction
Browse by: Latest US Patents, China's latest patents, Technical Efficacy Thesaurus, Application Domain, Technology Topic, Popular Technical Reports.
© 2024 PatSnap. All rights reserved.Legal|Privacy policy|Modern Slavery Act Transparency Statement|Sitemap|About US| Contact US: help@patsnap.com