Multi-period steel rail damage trend prediction method based on data mining
A data mining and trend forecasting technology, applied in data mining, structured data retrieval, digital data processing, etc., to achieve the effect of saving regular maintenance costs
- Summary
- Abstract
- Description
- Claims
- Application Information
AI Technical Summary
Problems solved by technology
Method used
Image
Examples
Embodiment Construction
[0023] The present invention will be further described below through specific embodiments in conjunction with the accompanying drawings. These embodiments are only used to illustrate the present invention, and are not intended to limit the protection scope of the present invention.
[0024] Such as figure 1 As shown, the specific implementation steps of the multi-period rail damage trend prediction method based on data mining described in the present invention are as follows:
[0025] S1, using a convolutional neural network to establish a location marker signal.
[0026] S11, combined with the original data of A-ultrasound and B-ultrasound, using a deep learning model to identify position marks such as hole joints, thermite welds, factory welding, on-site welding, guide holes, screw holes, etc., and establish position mark signals. The artificially marked lesion A was viewed as a 12-channel binary matrix superimposed into an image. Such as figure 2 As shown, the neural ne...
PUM
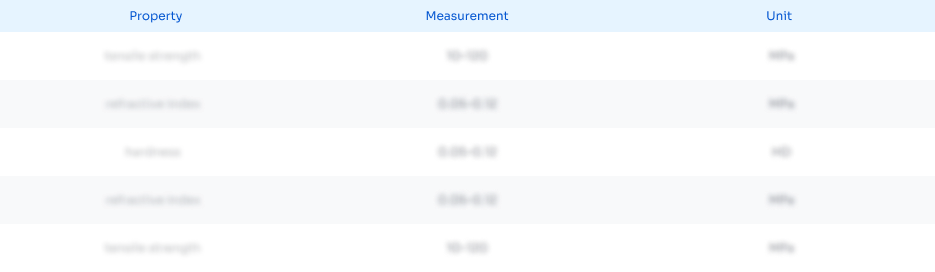
Abstract
Description
Claims
Application Information
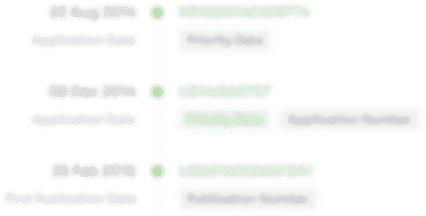
- R&D
- Intellectual Property
- Life Sciences
- Materials
- Tech Scout
- Unparalleled Data Quality
- Higher Quality Content
- 60% Fewer Hallucinations
Browse by: Latest US Patents, China's latest patents, Technical Efficacy Thesaurus, Application Domain, Technology Topic, Popular Technical Reports.
© 2025 PatSnap. All rights reserved.Legal|Privacy policy|Modern Slavery Act Transparency Statement|Sitemap|About US| Contact US: help@patsnap.com