Deep neural network target detection method based on multi-scale receptive field feature fusion
A deep neural network and feature fusion technology, applied in the field of image target detection, can solve the problems of insufficient target positioning capabilities of different scales, and achieve the effect of improving effect, improving performance, and improving network performance
- Summary
- Abstract
- Description
- Claims
- Application Information
AI Technical Summary
Problems solved by technology
Method used
Image
Examples
Embodiment Construction
[0027] The patent will be further described below in conjunction with the accompanying drawings and specific examples.
[0028] This patent can be applied to image object detection tasks, but is not limited to this task. Deep convolutional neural networks based on multi-scale receptive field feature fusion can be used to solve many tasks in applicable scenarios such as semantic segmentation and image classification. image 3 An example of the application of the deep convolutional neural network based on multi-scale receptive field feature fusion of the present invention for image target detection is described, and the implementation of this patent for image target detection tasks is introduced here.
[0029] Applying the present invention to image target detection tasks mainly includes three steps: collecting images and preparing data sets; designing and training a deep convolutional neural network based on fusion of multi-scale receptive field features; testing / applying a det...
PUM
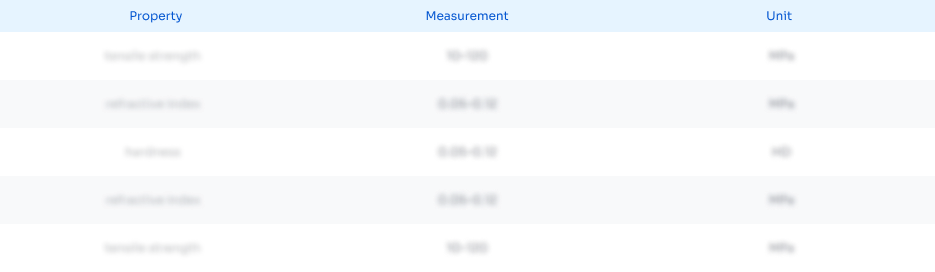
Abstract
Description
Claims
Application Information
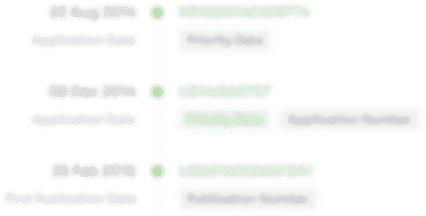
- R&D Engineer
- R&D Manager
- IP Professional
- Industry Leading Data Capabilities
- Powerful AI technology
- Patent DNA Extraction
Browse by: Latest US Patents, China's latest patents, Technical Efficacy Thesaurus, Application Domain, Technology Topic, Popular Technical Reports.
© 2024 PatSnap. All rights reserved.Legal|Privacy policy|Modern Slavery Act Transparency Statement|Sitemap|About US| Contact US: help@patsnap.com