Deep learning intelligent modulation identification method based on cyclic spectrum estimation
A technology of deep learning and modulation recognition, applied in character and pattern recognition, computing, computer components, etc., can solve problems such as high complexity and many input parameters, and achieve the effect of improving performance and reducing time complexity
- Summary
- Abstract
- Description
- Claims
- Application Information
AI Technical Summary
Problems solved by technology
Method used
Image
Examples
Embodiment 1
[0037] Such as figure 1 As shown, the deep learning intelligent modulation recognition method based on cyclic spectrum estimation includes the following steps:
[0038] S1: Generate a modulated signal according to the carrier frequency and symbol rate;
[0039] S2: Estimate the cyclic spectrum of the modulated signal, and extract the cross-sectional view of the cyclic spectrum function;
[0040] S3: Use the cross-sectional image as a feature to train a deep neural network;
[0041] S4: Use the deep neural network to identify the modulation mode of the unknown signal.
[0042] More specifically, the step S1 is specifically: when the frequency f is used s =16000Hz, carrier frequency f c =5000Hz, symbol rate f d = 1200Hz, generate 2ASK, 2FSK, BPSK, QPSK four modulation signals, and propagate the four modulation signals through the Gaussian channel.
[0043] Wherein, in the step S2, the calculation formula of the cyclic spectrum estimation is specifically:
[0044]
[0045] among them, t Me...
Embodiment 2
[0056] More specifically, on the basis of Embodiment 1, the method of the present invention is further described by taking the identification of four modulation signals of 2ASK, 2FSK, BPSK, and QPSK as an example.
[0057] In the specific implementation process, the frequency f s =16000Hz, carrier frequency f c =5000Hz, symbol rate f d = 1200Hz, generate 2ASK, 2FSK, BPSK, QPSK four modulation signals, and propagate the four modulation signals through the Gaussian channel; calculate the cyclic spectrum of the four signals, and extract the cross section of the cyclic spectrum function And put the corresponding label as the original training data.
[0058] In the specific implementation process, the calculation formula for cyclic spectrum estimation is specifically:
[0059]
[0060] among them, t Means time average statistics; Represents a cross-sectional view of the cyclic spectrum function.
[0061] More specifically, the time smoothing method is used to For estimation, the specif...
PUM
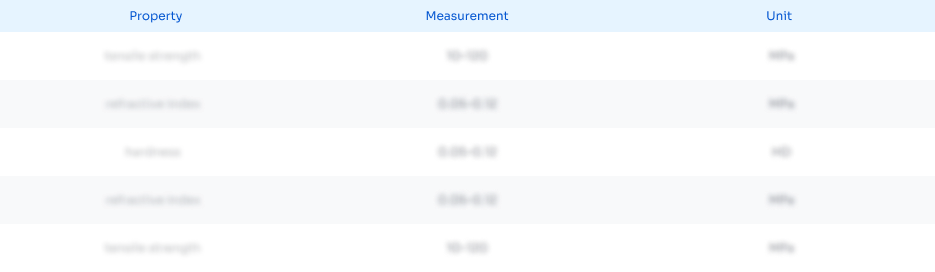
Abstract
Description
Claims
Application Information
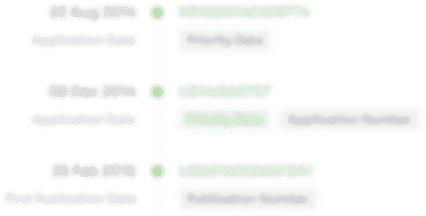
- R&D Engineer
- R&D Manager
- IP Professional
- Industry Leading Data Capabilities
- Powerful AI technology
- Patent DNA Extraction
Browse by: Latest US Patents, China's latest patents, Technical Efficacy Thesaurus, Application Domain, Technology Topic, Popular Technical Reports.
© 2024 PatSnap. All rights reserved.Legal|Privacy policy|Modern Slavery Act Transparency Statement|Sitemap|About US| Contact US: help@patsnap.com