Electric energy quality prediction method based on similar days and improved LSTM
A power quality monitoring and power quality technology, applied in neural learning methods, character and pattern recognition, biological neural network models, etc., can solve problems such as difficult nonlinear data fitting, complex calculation of combined models, and small degree of dispersion. Achieve the effect of reducing time consumption, avoiding information interference, and improving accuracy
- Summary
- Abstract
- Description
- Claims
- Application Information
AI Technical Summary
Problems solved by technology
Method used
Image
Examples
Embodiment Construction
[0053]The present invention will be described in detail below in conjunction with the accompanying drawings and specific embodiments. Apparently, the described embodiments are some, not all, embodiments of the present invention. Based on the embodiments of the present invention, all other embodiments obtained by persons of ordinary skill in the art without making creative efforts shall fall within the protection scope of the present invention.
[0054] Such as figure 1 Shown, the present invention relates to a kind of power quality prediction method based on similar day and improved LSTM, comprises the following steps:
[0055] Step 1. Based on the power grid power quality monitoring platform, collect the power quality steady-state index data of a certain monitoring point in a certain region and the meteorological data of the region for a period of time.
[0056] Step 2: Use Kernel Principal Component Analysis (KPCA) to perform feature dimensionality reduction on the meteoro...
PUM
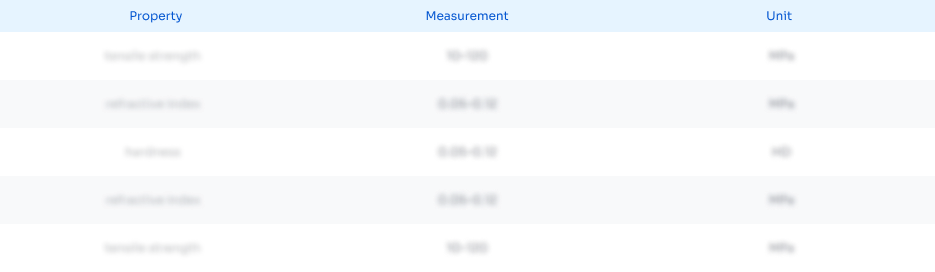
Abstract
Description
Claims
Application Information
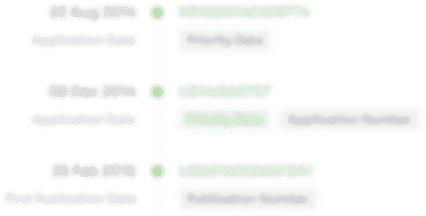
- R&D Engineer
- R&D Manager
- IP Professional
- Industry Leading Data Capabilities
- Powerful AI technology
- Patent DNA Extraction
Browse by: Latest US Patents, China's latest patents, Technical Efficacy Thesaurus, Application Domain, Technology Topic, Popular Technical Reports.
© 2024 PatSnap. All rights reserved.Legal|Privacy policy|Modern Slavery Act Transparency Statement|Sitemap|About US| Contact US: help@patsnap.com