Propagation-free model wireless network planning method based on machine learning
A machine learning and dissemination model technology, applied in network planning, wireless communication, electrical components, etc., can solve the problems that the experience dissemination model is no longer applicable, and the base station consumes a lot of manpower and material resources, so as to reduce deployment costs, reduce complexity, and improve The effect of accuracy
- Summary
- Abstract
- Description
- Claims
- Application Information
AI Technical Summary
Problems solved by technology
Method used
Image
Examples
Embodiment Construction
[0062] The implementation cases of the present invention are described in detail in conjunction with the accompanying drawings.
[0063] Considering the geographic constraints in practical applications and the constraints on operating parameters of base stations, the present invention implements a low-cost base station deployment solution based on machine learning and greedy methods. First, a large amount of relevant information including user measurements and configuration parameters of base stations in the real world is extracted. The collected data is then fed into the data processing module for further training. We train the regression model using some machine learning algorithm and perform hyperparameter optimization by grid search method. Second, the optimal parameters of multiple base stations are determined by utilizing the training model and an online optimization algorithm.
[0064] attached figure 1 It is a screenshot of the data type that needs to be extracted i...
PUM
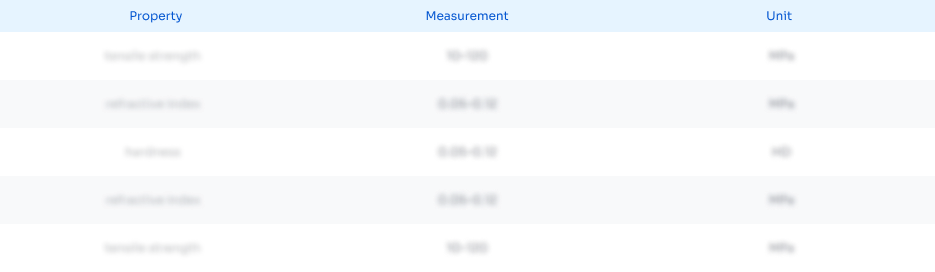
Abstract
Description
Claims
Application Information
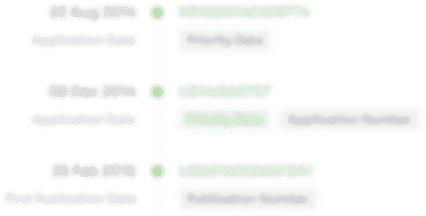
- R&D Engineer
- R&D Manager
- IP Professional
- Industry Leading Data Capabilities
- Powerful AI technology
- Patent DNA Extraction
Browse by: Latest US Patents, China's latest patents, Technical Efficacy Thesaurus, Application Domain, Technology Topic, Popular Technical Reports.
© 2024 PatSnap. All rights reserved.Legal|Privacy policy|Modern Slavery Act Transparency Statement|Sitemap|About US| Contact US: help@patsnap.com