A Road Scene Semantic Segmentation Method Effectively Fusion of Neural Network Features
A neural network and semantic segmentation technology, applied in neural learning methods, biological neural network models, scene recognition, etc., can solve the problem that simple fusion of low-level and high-level features is not effective, so as to improve the accuracy of semantic segmentation and information loss The effect of improving and enhancing robustness
- Summary
- Abstract
- Description
- Claims
- Application Information
AI Technical Summary
Problems solved by technology
Method used
Image
Examples
Embodiment Construction
[0042] The present invention will be further described in detail below in conjunction with the accompanying drawings and embodiments.
[0043] The present invention proposes a road scene semantic segmentation method that effectively integrates neural network features, which includes two processes, a training phase and a testing phase.
[0044] The specific steps of the described training phase process are:
[0045] Step 1_1: Select Q original road scene images and the real semantic segmentation images corresponding to each original road scene image, and form a training set, and record the qth original road scene image in the training set as {I q (i,j)}, combine the training set with {I q (i, j)} corresponding to the real semantic segmentation image is denoted as Then, the existing one-hot encoding technology (one-hot) is used to process the real semantic segmentation images corresponding to each original road scene image in the training set into 12 one-hot encoded images. ...
PUM
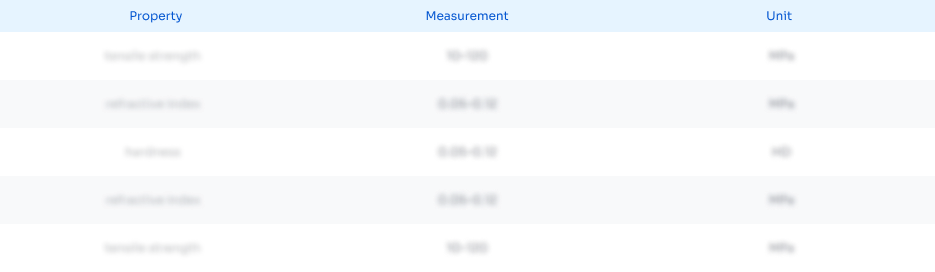
Abstract
Description
Claims
Application Information
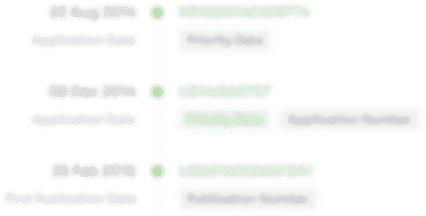
- R&D Engineer
- R&D Manager
- IP Professional
- Industry Leading Data Capabilities
- Powerful AI technology
- Patent DNA Extraction
Browse by: Latest US Patents, China's latest patents, Technical Efficacy Thesaurus, Application Domain, Technology Topic, Popular Technical Reports.
© 2024 PatSnap. All rights reserved.Legal|Privacy policy|Modern Slavery Act Transparency Statement|Sitemap|About US| Contact US: help@patsnap.com