Magnetic resonance image reconstruction method based on regularized depth image prior method
A technology for magnetic resonance images and depth images, applied in image enhancement, image analysis, image data processing, etc., can solve the problems of lack and difficulty in obtaining medical magnetic resonance image data sets, and achieve accelerated reconstruction, high computing efficiency, and reconstructed images short time effect
- Summary
- Abstract
- Description
- Claims
- Application Information
AI Technical Summary
Problems solved by technology
Method used
Image
Examples
Embodiment Construction
[0071] The present invention will be described in detail below in conjunction with the accompanying drawings and examples.
[0072] Such as figure 1 Shown, the specific implementation steps of the present invention are as follows:
[0073] (1) Construct a neural network model;
[0074] (2) Construct a loss function containing regular terms; including mean square error, l 1 Norm and Laplacian three parts, where l 1 Norm and Laplacian are used as image regularization items to provide image prior information for the network, and minimize the loss function during iteration to optimize network parameters;
[0075] (3) Using the pre-set undersampling template to obtain part of the k-space data;
[0076] (4) Obtaining a reconstruction reference image: performing inverse Fourier transform directly after zero-filling part of the acquired k-space data, and obtaining a spatial domain degraded magnetic resonance image as a reconstruction reference image;
[0077] (5) Constructing th...
PUM
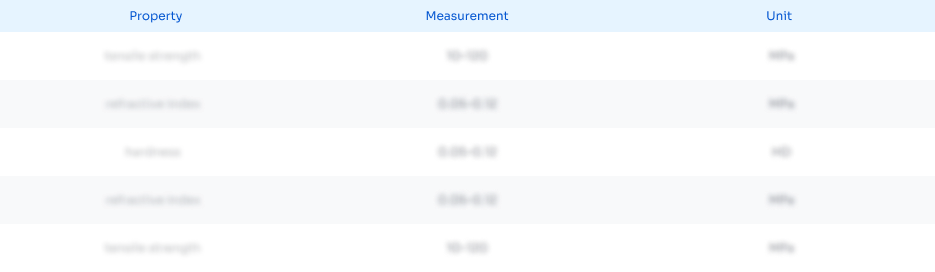
Abstract
Description
Claims
Application Information
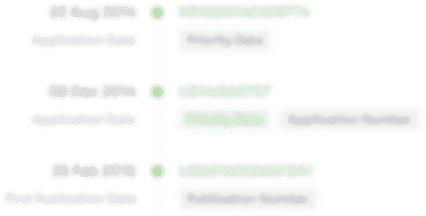
- R&D
- Intellectual Property
- Life Sciences
- Materials
- Tech Scout
- Unparalleled Data Quality
- Higher Quality Content
- 60% Fewer Hallucinations
Browse by: Latest US Patents, China's latest patents, Technical Efficacy Thesaurus, Application Domain, Technology Topic, Popular Technical Reports.
© 2025 PatSnap. All rights reserved.Legal|Privacy policy|Modern Slavery Act Transparency Statement|Sitemap|About US| Contact US: help@patsnap.com