Detailed classifying system for sleep incubation period
A technology for fine-grained classification and light sleep, applied in the sleep field, can solve the problems that ordinary consumers cannot use conveniently, multi-leads are sensitive to paste, and sensors are easy to fall off, so as to ensure the stability of hardware operation, facilitate wear and use, and improve data quality. The effect of accuracy
- Summary
- Abstract
- Description
- Claims
- Application Information
AI Technical Summary
Problems solved by technology
Method used
Image
Examples
Embodiment 2
[0097] Compared with the first embodiment, this embodiment provides another decision tree structure.
[0098] In actual operation, at the first node, the threshold Num-LCZ-Threshold is set. When Num-LCZ > Num-LCZ-Threshold, the single-frame data packet is determined as an artificial artifact, and is sent to the processor by the processor. The external output is the sleep state obtained when processing the last single frame of data packet, otherwise, it transfers to the second node. When artificial artifacts appear, the number of times that the waveform crosses the x-axis in each waveform graph will increase significantly. By comparing the value of the parameter Num-LCZ with the corresponding threshold Num-LCZ-Threshold to determine whether artificial artifacts are generated, specifically , when Num-LCZ>Num-LCZ-Threshold, the single-frame data packet is determined to be an artificial artifact, the single-frame data packet is invalid, and the sleep state obtained when the previo...
Embodiment 3
[0101] Compared with the first embodiment, this embodiment provides another decision tree structure.
[0102] At the second node, the threshold value BVS-Threshold is set. When BVS>BVS-Threshold, the processor determines that the user is in the sleep-onset latency or rapid eye movement period, and transfers to the fifth node. Otherwise, the processor determines that the user is in light sleep Period, light sleep period or deep sleep period, transfer to the third node. When BVS>BVS-Threshold, it means that the amplitude ratio of the alpha wave signal or beta wave signal in the single-frame data packet is stronger, which means that the user's brain is active and in the REM state or the awake state. On the contrary, it means that the amplitude ratio of the alpha wave signal and the beta wave signal in the single-frame data packet is weaker, which means that the user's brain is inactive and is in a light sleep state, a light sleep state, or a deeper sleep state.
[0103] Other fe...
Embodiment 4
[0105] Compared with the first embodiment, this embodiment provides another decision tree structure.
[0106] Set the threshold TVB-Threshold and Num-EOG-Threshold. When TVB>TVA-Threshold and Num-EOG>Num-EOG-Threshold, the processor determines that the user is in the rapid eye movement period, the system stops working, and the single The frame data packet is recorded as a sleep frame data packet, otherwise, the processor determines that the user is in the sleep latency period, and transfers to the sixth node. When TVB>TVB-Threshold, and Num-EOG>Num-EOG-Threshold, the processor determines that the user's brain thinking is in the REM state with theta wave components and irregular eye movements, ends the sleep detection, and records the single The frame data packet is a sleep frame data packet. On the contrary, it means that the user's brain is in a awake state, and it is transferred to the T6 node for further analysis and processing.
[0107] Other features of the sleep-onset ...
PUM
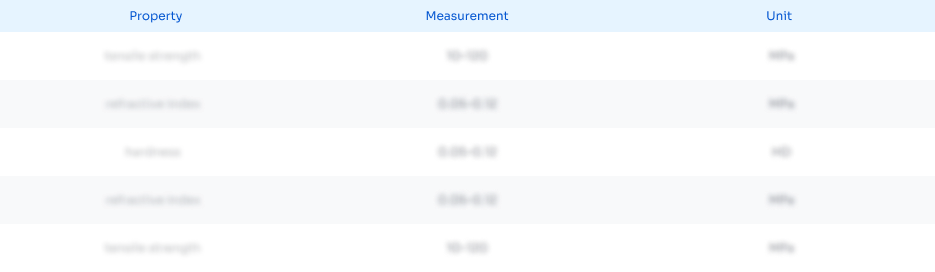
Abstract
Description
Claims
Application Information
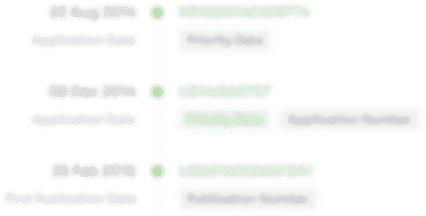
- R&D Engineer
- R&D Manager
- IP Professional
- Industry Leading Data Capabilities
- Powerful AI technology
- Patent DNA Extraction
Browse by: Latest US Patents, China's latest patents, Technical Efficacy Thesaurus, Application Domain, Technology Topic, Popular Technical Reports.
© 2024 PatSnap. All rights reserved.Legal|Privacy policy|Modern Slavery Act Transparency Statement|Sitemap|About US| Contact US: help@patsnap.com