Mobile robot collision avoidance planning method based on deep reinforcement learning in static environment
A mobile robot, static environment technology, applied in the field of mobile robot navigation, can solve the problems of unsuitable dynamic unknown environment, large limitations, etc., to achieve rich exploration strategies, smooth obstacle avoidance trajectory, and high portability.
- Summary
- Abstract
- Description
- Claims
- Application Information
AI Technical Summary
Problems solved by technology
Method used
Image
Examples
Embodiment Construction
[0034] The present invention will be further described below in conjunction with accompanying drawings and cases.
[0035] The invention discloses a mobile robot collision avoidance planning method based on deep reinforcement learning in a static environment, which can be used for effective obstacle avoidance when a mobile robot works in a complex static obstacle environment including a loop-shaped obstacle environment. The present invention performs corresponding data processing on the basis of the original data collected by the laser range finder, takes the processed data as the state S of the A3C algorithm, constructs the corresponding A3C-LSTM neural network, takes the state S as the network input, and passes the A3C Algorithm, the neural network outputs the corresponding parameters, and uses the relevant parameters to select the actions performed by the mobile robot at each step through the normal distribution. The overall obstacle avoidance algorithm flow chart is as foll...
PUM
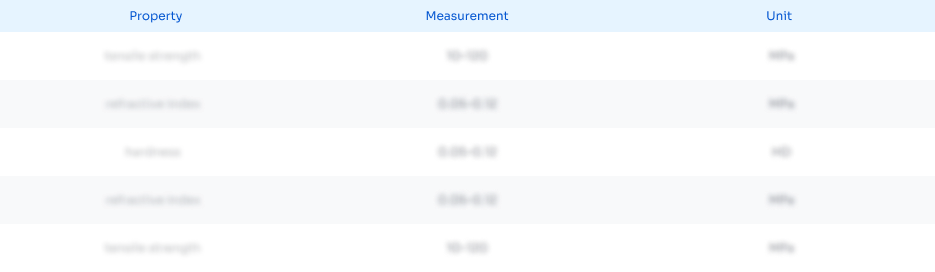
Abstract
Description
Claims
Application Information
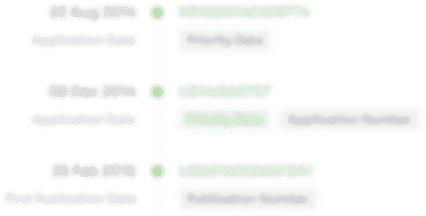
- R&D
- Intellectual Property
- Life Sciences
- Materials
- Tech Scout
- Unparalleled Data Quality
- Higher Quality Content
- 60% Fewer Hallucinations
Browse by: Latest US Patents, China's latest patents, Technical Efficacy Thesaurus, Application Domain, Technology Topic, Popular Technical Reports.
© 2025 PatSnap. All rights reserved.Legal|Privacy policy|Modern Slavery Act Transparency Statement|Sitemap|About US| Contact US: help@patsnap.com