Cloth defect classification method based on LBP features and convolutional neural network
A convolutional neural network and defect classification technology, applied in the field of fabric defect classification based on LBP features and convolutional neural network, can solve the problems of high false detection rate and missed detection rate, detection efficiency of detection algorithm and low detection accuracy, etc. Achieve the effect of improving accuracy, high defect detection accuracy, and simple algorithm implementation
- Summary
- Abstract
- Description
- Claims
- Application Information
AI Technical Summary
Problems solved by technology
Method used
Image
Examples
Embodiment Construction
[0030] In order to make the technical solutions and advantages of the present invention more clear, the technical solutions in the embodiments of the present invention are clearly and completely described below in conjunction with the drawings in the embodiments of the present invention:
[0031] A cloth defect classification method based on LBP features and convolutional neural network of the present invention, figure 1 It is a flow chart of the defect classification method of the present invention, figure 2 The flow chart of the steps for defect classification, the specific implementation steps are as follows:
[0032] Step 1: Collect multiple images of cloth samples;
[0033] Step 2: In order for the support vector machine model to be able to finally classify the defects, it is divided into three folders: normal image, hole hole image and plasma spot image, and store the image name and the corresponding number 0-3; the label type is: normal is the number 0, the hole is t...
PUM
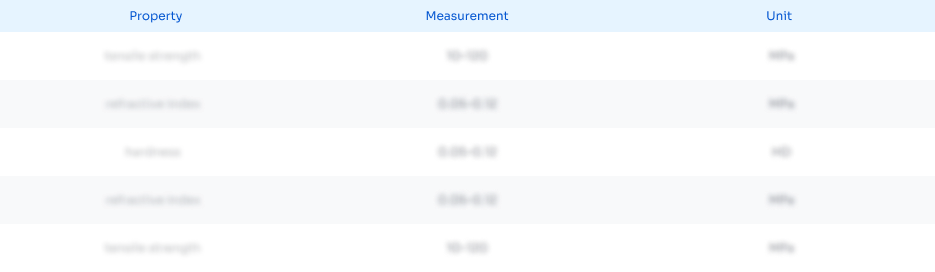
Abstract
Description
Claims
Application Information
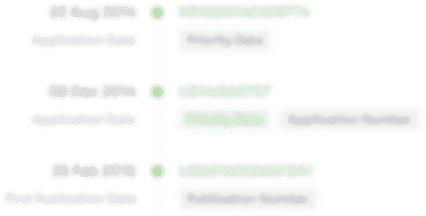
- R&D Engineer
- R&D Manager
- IP Professional
- Industry Leading Data Capabilities
- Powerful AI technology
- Patent DNA Extraction
Browse by: Latest US Patents, China's latest patents, Technical Efficacy Thesaurus, Application Domain, Technology Topic, Popular Technical Reports.
© 2024 PatSnap. All rights reserved.Legal|Privacy policy|Modern Slavery Act Transparency Statement|Sitemap|About US| Contact US: help@patsnap.com