Soft measurement method and system for vine copula correlation description based on Hamiltonian Monte Carlo sampling
A soft measurement and correlation technology, applied in the field of soft measurement, can solve problems affecting the effect of soft measurement, information loss, etc.
- Summary
- Abstract
- Description
- Claims
- Application Information
AI Technical Summary
Problems solved by technology
Method used
Image
Examples
Embodiment 1
[0126] The present invention discloses a soft-sensing method based on the vine copula correlation description of Hamiltonian Monte Carlo sampling, and the specific steps are as follows:
[0127] [Step S1]: Select appropriate auxiliary variables for the soft sensor model according to the actual industrial production situation and expert knowledge
[0128] [Step S2]: Use the monotone transformation method to obtain the transformed data conforming to copula modeling, and calculate the average variance of the target variable of the training data:
[0129] See formula (1) for the zero-mean standardization of the original data
[0130]
[0131] in,
[0132] x i is the variable before transformation, X i ’ is the variable after zero-mean standardization, mean(X i ) is the variable X i mean, sd(X i ) is the variable X i The standard deviation of , d is the dimension of the vector X. Define the monotone transformation form, see formula (2):
[0133] Z i =(1-α i )X i '+α ...
Embodiment 2
[0186] The description of the following examples will help to understand the present invention, but does not limit the content of the present invention. see figure 2 , the present embodiment realizes the prediction (PER) of the degree of ethylene cracking in the ethylene cracking process. The data of this implementation example comes from the SRT-III model ethylene cracking furnace, and the prediction target is the ethylene cracking rate, which is determined by PER (propylene / ethylene ratio) Said that 500 sets of data under normal working conditions were selected, 400 sets were used to train the copula model, and 100 sets were used for testing.
[0187] (1) According to the prior information, four auxiliary variables are selected: the average outlet temperature of the cracking furnace x 1 , the density x of the pyrolysis feedstock 2 , total feed x 3 and steam hydrocarbon ratio x 4 . The target variable y is the lysis depth index PER.
[0188] (2) Data preprocessing: sta...
Embodiment 3
[0198] see Figure 4 , this embodiment realizes the prediction of acetylene concentration in the acetylene hydrogenation reactor. The data of this implementation example comes from the acetylene hydrogenation process, and the prediction target is the acetylene concentration. 250 sets of data in normal working conditions are selected, and 200 sets are used for training copula model, 50 for testing.
[0199] (1) According to prior information, three auxiliary variables are selected: C2 component feed rate x 1 , hydrogen feed rate x 2 , the temperature difference between inlet and outlet x 3 , the leading variable is the acetylene concentration y.
[0200] (2) Data preprocessing: standardize the zero mean value of the training samples, and select the last one-dimensional auxiliary variable x as the reference variable 3 , use the Pearson correlation coefficient method to perform monotone transformation, and obtain the transformed data [z 1 ,z 2 ,z 3 ,z v ].
[0201] (3) U...
PUM
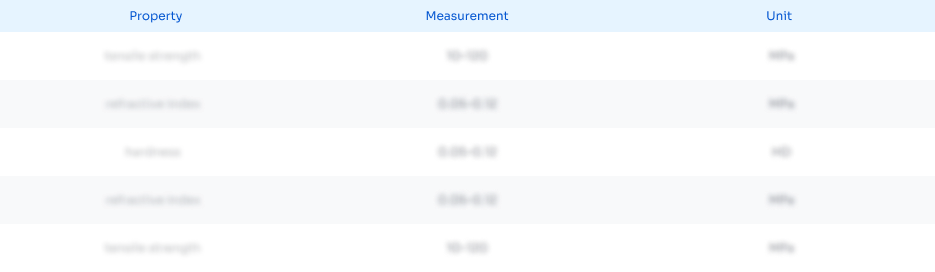
Abstract
Description
Claims
Application Information
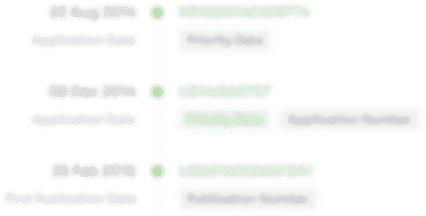
- R&D Engineer
- R&D Manager
- IP Professional
- Industry Leading Data Capabilities
- Powerful AI technology
- Patent DNA Extraction
Browse by: Latest US Patents, China's latest patents, Technical Efficacy Thesaurus, Application Domain, Technology Topic, Popular Technical Reports.
© 2024 PatSnap. All rights reserved.Legal|Privacy policy|Modern Slavery Act Transparency Statement|Sitemap|About US| Contact US: help@patsnap.com