Intelligent metallographic detection rating method and system based on deep learning
A technology of metallographic detection and deep learning, applied in image data processing, image enhancement, instruments, etc., can solve problems such as lack of universality and transplantability, inability to grade metal raw materials, and accuracy rate cannot be guaranteed, etc., to achieve Avoid multi-step operations, avoid classification calculations, and avoid cumbersome and unstable effects
- Summary
- Abstract
- Description
- Claims
- Application Information
AI Technical Summary
Problems solved by technology
Method used
Image
Examples
Embodiment approach
[0046] figure 1 Shown is a preferred implementation of the deep learning-based intelligent metallographic detection and rating method of the present invention. The deep learning-based intelligent metallographic detection and rating method includes the following steps:
[0047] Image collection: collect metal sample images;
[0048] Build an improved fully convolutional neural network: improve on the basis of the U-net fully convolutional neural network, and obtain the construction of an improved fully convolutional neural network;
[0049] Image Segmentation: The collected metal sample image is automatically segmented through the improved full convolutional neural network to obtain a metallographic segmentation map;
[0050] Image classification: automatically grade and classify the obtained metallographic segmentation images through the deep neural network;
[0051] Result Display: Display the results of segmentation and rating classification.
[0052] The image collection i...
PUM
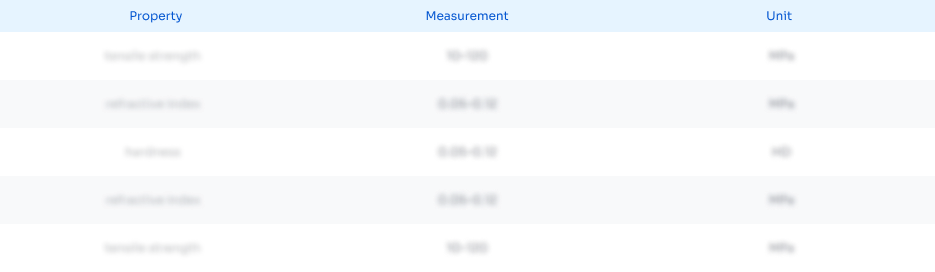
Abstract
Description
Claims
Application Information
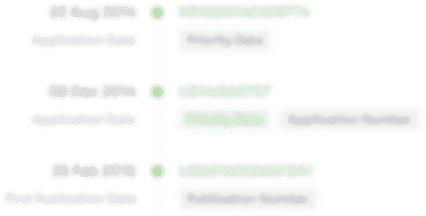
- R&D Engineer
- R&D Manager
- IP Professional
- Industry Leading Data Capabilities
- Powerful AI technology
- Patent DNA Extraction
Browse by: Latest US Patents, China's latest patents, Technical Efficacy Thesaurus, Application Domain, Technology Topic, Popular Technical Reports.
© 2024 PatSnap. All rights reserved.Legal|Privacy policy|Modern Slavery Act Transparency Statement|Sitemap|About US| Contact US: help@patsnap.com