Single cell transcriptome missing value filling method based on deep hybrid network
A hybrid network and single-cell technology, which is applied in the field of single-cell transcriptome deletion filling, can solve the problems of large computing resources, unreliable data interpretation, and inability to use single-cell transcriptome data universally, so as to improve reliability, reduce occupancy, Guaranteed versatility
- Summary
- Abstract
- Description
- Claims
- Application Information
AI Technical Summary
Problems solved by technology
Method used
Image
Examples
Embodiment 1
[0055] like figure 1 As shown, a single-cell transcriptome missing value filling method based on a deep hybrid network includes the following steps:
[0056] S1: Preprocess the single-cell sequencing data to obtain the expression matrix;
[0057] S2: Standardize the expression matrix to obtain the initial expression matrix;
[0058] S3: Build a hybrid model based on deep learning, including two parts: autoencoder and cyclic neural network;
[0059] S4: Input the initial expression matrix into the autoencoder for dimensionality reduction processing to obtain a dimensionality-reduced feature matrix and a reconstructed expression matrix;
[0060] S5: Input the dimensionality-reduced feature matrix into the recurrent neural network, predict the expression values of all genes, and obtain the corresponding predicted expression matrix;
[0061] S6: Using the predicted expression matrix obtained in step S5 as the input of the autoencoder, repeating step S4 and step S5 until the p...
Embodiment 2
[0065] More specifically, such as figure 2 As shown, the step S1 specifically includes the following steps:
[0066] S11: Use the existing library construction method to obtain the processed cells, perform sequencing to obtain sequence data, and the file format, such as Fastq;
[0067] S12: using mapping software, such as Tophat2, to map the sequence data;
[0068] S13: using data splitting software, such as UMI-tools, to divide the mapped sequence data by cells to obtain sequence splitting data;
[0069] S14: Use quantitative software, such as FeatureCounts, to quantify the mapped and divided results to obtain a gene × cell expression matrix.
[0070] More specifically, the step S2 is specifically:
[0071] The expression matrix is normalized according to the library size ls of each cell to eliminate the effect of library size, where, for the gene expression value vector C of cell c c The standardized formula for is:
[0072]
[0073] Among them, sf represents the ...
Embodiment 3
[0085] More specifically, such as Figure 4As shown, in the application process of the hybrid model, the single-cell data is input into the hybrid model by using non-blocking multi-process block random read data; the specific process is:
[0086] Enter the storage address of the single-cell data file, which meets any type of access matrix and read in blocks;
[0087] According to the storage address, read the dimension information of the single-cell transcriptome matrix stored in the file, including the number of cells and the number of genes, and enter the corresponding cell name and gene name;
[0088] Divide all cells into multiple data clusters in order, and mark each data cluster with a serial number, and all cluster serial numbers are used as a serial number pool;
[0089] Create a copy based on the serial number pool, randomly extract a certain number of cluster serial numbers without replacement each time, and extract the data set. If the copy data is extracted, a new...
PUM
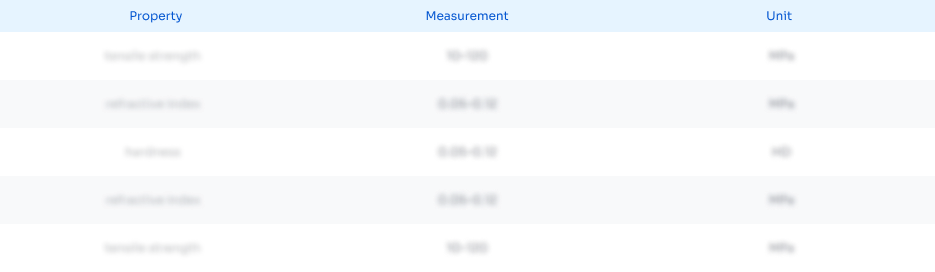
Abstract
Description
Claims
Application Information
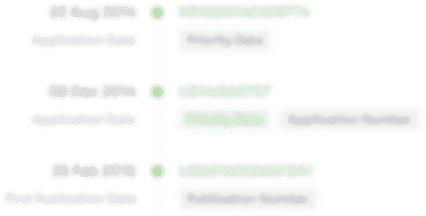
- R&D Engineer
- R&D Manager
- IP Professional
- Industry Leading Data Capabilities
- Powerful AI technology
- Patent DNA Extraction
Browse by: Latest US Patents, China's latest patents, Technical Efficacy Thesaurus, Application Domain, Technology Topic.
© 2024 PatSnap. All rights reserved.Legal|Privacy policy|Modern Slavery Act Transparency Statement|Sitemap