Numerical control equipment remote diagnosis system based on neural network
A neural network and remote diagnosis technology, which is applied in the field of remote diagnosis system of numerical control equipment, can solve the problems of low maintenance efficiency and long detection time of numerical control machine tools, and achieve the effect of fast processing efficiency, improved detection efficiency and success rate, and fast processing speed.
- Summary
- Abstract
- Description
- Claims
- Application Information
AI Technical Summary
Problems solved by technology
Method used
Image
Examples
Embodiment
[0037] A neural network-based remote diagnosis system for CNC equipment, basically as figure 1 As shown, including the server, the server includes the following modules:
[0038] Parameter collection module: used to obtain model parameters and operating parameters of CNC machine tools;
[0039] Preliminary diagnosis module: used for preliminary diagnosis of the CNC machine tool according to the operating parameters, analyzing whether the CNC machine tool is normal or faulty, and marking the operating parameters as faulty operating parameters when the CNC machine tool is initially diagnosed as faulty;
[0040] In-depth diagnosis module: used to import fault operating parameters into the established neural network fault diagnosis model for diagnosis, and output fault diagnosis results; the diagnosis results include fault types of CNC machine tools normal and CNC machine faults; neural network fault diagnosis model Construct based on BP neural network, collect data sample sets f...
Embodiment 2
[0051] The difference between the embodiment and the first embodiment is that the construction method of the shown neural network fault diagnosis model is as follows,
[0052] Step 1: Determine the input and output vectors:
[0053] According to the construction principle of the Boolean matrix, it is defined that in the fault diagnosis, there are m characteristic parameters, that is, the input characteristic vector P=(s 1 ,s 2 ,...,s m ), there are n fault types to be identified, that is, the output feature vector Q=(r 1 ,r 2 ,...,r n );
[0054] Step 2: Select the number of network layers: use a three-layer BP neural network, which are input layer, hidden layer, and output layer; according to the input feature vector and output feature vector described in step 1, determine the number of neurons in the input layer as a , where a=m, the number of neurons in the output layer is b, where b=n;
[0055] Step 3: Calculate the number of neurons in the hidden layer: the number ...
PUM
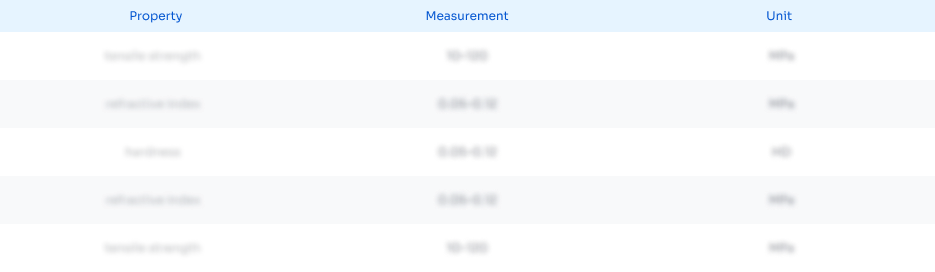
Abstract
Description
Claims
Application Information
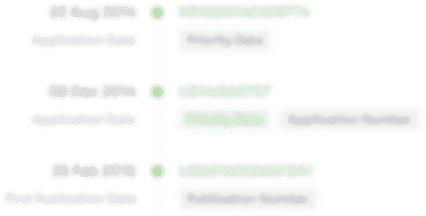
- R&D Engineer
- R&D Manager
- IP Professional
- Industry Leading Data Capabilities
- Powerful AI technology
- Patent DNA Extraction
Browse by: Latest US Patents, China's latest patents, Technical Efficacy Thesaurus, Application Domain, Technology Topic, Popular Technical Reports.
© 2024 PatSnap. All rights reserved.Legal|Privacy policy|Modern Slavery Act Transparency Statement|Sitemap|About US| Contact US: help@patsnap.com