New energy lithium battery surface defect detection method based on adaptive deep learning
A deep learning and defect detection technology, applied in the fields of computer vision and deep learning, can solve problems such as inability to achieve optimal performance, inability to call light sources, complex system parameters, etc., to improve labeling efficiency and accuracy, improve defect detection efficiency, The effect of improving detection efficiency
- Summary
- Abstract
- Description
- Claims
- Application Information
AI Technical Summary
Problems solved by technology
Method used
Image
Examples
Embodiment 1
[0064] The new energy lithium battery surface defect detection method based on adaptive deep learning includes:
[0065] Step 1: Collect the grayscale image of the lithium battery surface, use a nonlinear operator to perform nonlinear mapping on the grayscale image of the lithium battery surface, and decouple the irradiation component and the reflection component:
[0066] ln(G(x,y)+1)=ln(I(x,y)+1)+ln(R(x,y)+1)
[0067] Among them, G is the grayscale image of the lithium battery surface, I is the irradiation component, R is the reflection component, and (x, y) are the pixel coordinates.
[0068] The grayscale image G of the lithium battery surface is acquired by an ordinary area scan camera. In the image acquisition, since the lithium battery is cylindrical, it is necessary to take images of the four directions of the lithium battery.
[0069] Fix the camera, drive the battery to rotate through the servo motor and the tray, and rotate 90 degrees each time, and obtain four im...
PUM
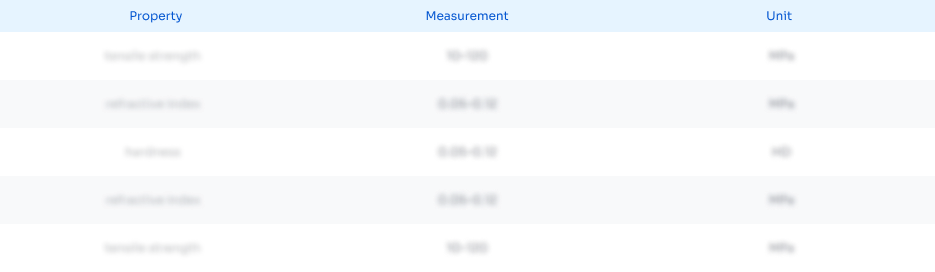
Abstract
Description
Claims
Application Information
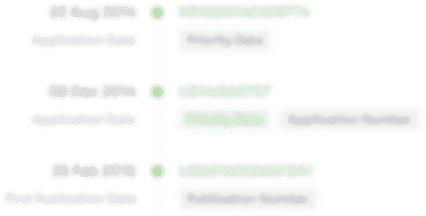
- R&D Engineer
- R&D Manager
- IP Professional
- Industry Leading Data Capabilities
- Powerful AI technology
- Patent DNA Extraction
Browse by: Latest US Patents, China's latest patents, Technical Efficacy Thesaurus, Application Domain, Technology Topic, Popular Technical Reports.
© 2024 PatSnap. All rights reserved.Legal|Privacy policy|Modern Slavery Act Transparency Statement|Sitemap|About US| Contact US: help@patsnap.com