Breast cancer image recognition method and system based on multi-stage and multi-feature deep fusion
An image recognition and multi-feature technology, applied in the field of information processing, can solve problems such as high complexity, time-consuming cost, easy loss of identification information, etc., to reduce the requirements of machine hardware configuration, reduce time cost and equipment cost, reduce Effect of Model Parameter Quantity
- Summary
- Abstract
- Description
- Claims
- Application Information
AI Technical Summary
Problems solved by technology
Method used
Image
Examples
Embodiment 1
[0083] Multi-stage multi-feature deep fusion refers to: 1) Using multiple complementary features, such as traditional features and deep learning features, there are four traditional features and three deep learning features. Because the feature extraction methods are different and heterogeneous, they describe mammography images from different visual angles; 2) There are several simple, easy and effective feature fusion methods: feature early fusion, feature fusion and feature post fusion. Each stage contributes to an improvement in the performance of the final breast cancer diagnosis.
[0084] Based on the idea of multi-stage and multi-feature deep fusion, a new DE-Ada* breast cancer diagnosis model is proposed, which includes four parts: image feature extraction, cross-modal pathological semantic mining, multi-feature fusion and breast cancer classification. Gist(G), SIFT(S), HOG(H), LBP(L), VGG16(V), ResNet(R), DenseNet(D) features of images are extracted from the perspect...
PUM
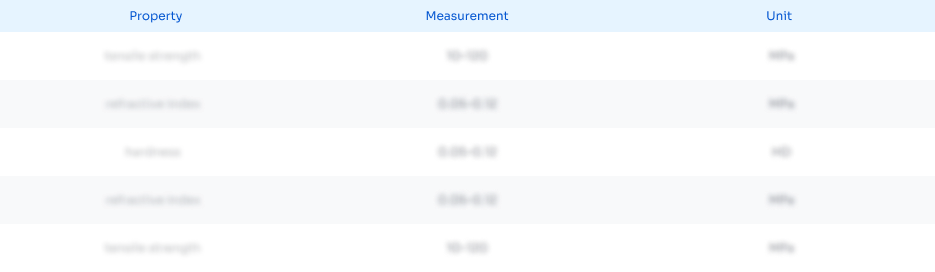
Abstract
Description
Claims
Application Information
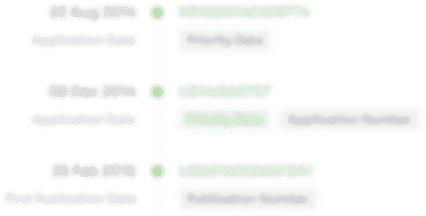
- Generate Ideas
- Intellectual Property
- Life Sciences
- Materials
- Tech Scout
- Unparalleled Data Quality
- Higher Quality Content
- 60% Fewer Hallucinations
Browse by: Latest US Patents, China's latest patents, Technical Efficacy Thesaurus, Application Domain, Technology Topic, Popular Technical Reports.
© 2025 PatSnap. All rights reserved.Legal|Privacy policy|Modern Slavery Act Transparency Statement|Sitemap|About US| Contact US: help@patsnap.com