Angle-dependent complex array error calibration method based on deep neural network
A deep neural network and angle-dependent technology, which is applied in the field of angle-dependent complex array error calibration, can solve problems such as angle-dependent complex array error calibration, and achieves reduced training data volume, good calibration performance, and small residual array errors. Effect
- Summary
- Abstract
- Description
- Claims
- Application Information
AI Technical Summary
Problems solved by technology
Method used
Image
Examples
Embodiment Construction
[0020] The specific implementation manners of the present invention will be described in further detail below in conjunction with the accompanying drawings. refer to figure 1 , the specific implementation steps of the present invention are as follows:
[0021] (S1): Place the array on the servo platform in the darkroom, and fix a corner reflector or radiation source in the far field of the array according to the active or passive working mode of the array, and collect darkroom data. Set the system parameters so that the signal-to-noise ratio of the array output baseband signal is as close as possible to the maximum value within the dynamic range. Rotate the servo so that the arrival angle of the radiation signal of the radiation source relative to the normal line of the array is θ from small to large1 ,θ 2 ,...,θ L , record the array output baseband signal x corresponding to each angle 1 ,x 2 ,...,x L , where x l , l=1,2,..., L is the M-dimensional complex vector, M is ...
PUM
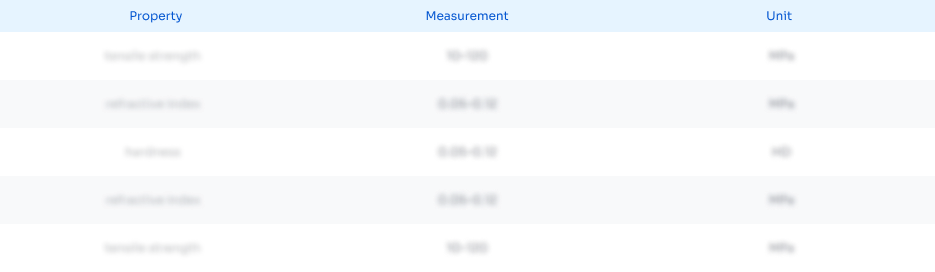
Abstract
Description
Claims
Application Information
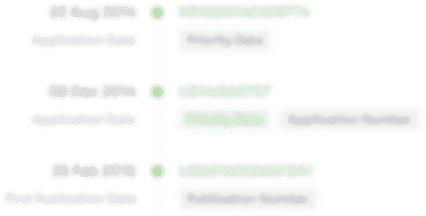
- R&D Engineer
- R&D Manager
- IP Professional
- Industry Leading Data Capabilities
- Powerful AI technology
- Patent DNA Extraction
Browse by: Latest US Patents, China's latest patents, Technical Efficacy Thesaurus, Application Domain, Technology Topic, Popular Technical Reports.
© 2024 PatSnap. All rights reserved.Legal|Privacy policy|Modern Slavery Act Transparency Statement|Sitemap|About US| Contact US: help@patsnap.com