Image-text double-coding implementation method and system based on CR2 neural network
A neural network and implementation method technology, applied in the field of image-text dual coding, can solve the problems of low level, difficult to obtain labeled data, stay in theoretical text expression, etc., to reduce the requirements of shooting technology, improve the matching efficiency, and meet the needs of use. The effect of experience
- Summary
- Abstract
- Description
- Claims
- Application Information
AI Technical Summary
Problems solved by technology
Method used
Image
Examples
Embodiment 1
[0078] The CR-based 2 The image-text double encoding method of neural network is as follows: figure 1 As shown, as a preferred embodiment, such as image 3 As shown, the method for obtaining an image represented by non-words in image information through an image information acquisition program provided by an embodiment of the present invention includes:
[0079] S201. Collect a plurality of image information in a predetermined area, and simultaneously collect location scene and time information corresponding to the images.
[0080] S202. Obtain target sample information, input the plurality of image information into the matching model that has been trained in advance, and match the image information with the target sample information to obtain the image information and the target sample information Information matching results.
[0081] S203, using the trained multi-layer convolutional neural network (CNN) to analyze the image information collected in the predetermined area...
Embodiment 2
[0091] The CR-based 2The implementation method of image-text double encoding of neural network is as follows: figure 1 shown, as Figure 4 As shown, as a preferred embodiment, the method for acquiring the text semantic encoding of the text information word representation through the text semantic acquisition program provided by the embodiment of the present invention includes:
[0092] S301. Obtain a natural language text related to the semantics of the text represented by the words of the text information, and perform text clipping, replacement of rare words, word segmentation, and keyword processing.
[0093] S302. Obtain training data with known long text, extract training features of the training data to construct a training feature vector set, perform unsupervised clustering on the training feature vector set, and obtain multiple training centroids.
[0094] S303. Obtain evaluation data from the long text to be evaluated through the text processing model, extract evalua...
Embodiment 3
[0101] The CR-based 2 The implementation method of image-text double encoding of neural network is as follows: figure 1 shown, as Figure 5 As shown, as a preferred embodiment, the method for associating image information and text semantic coding information through the image-text association program provided by the embodiment of the present invention includes:
[0102] S401, perform feature extraction and expression for image and text semantics, and respectively obtain feature vector spaces of semantic primitives in separate modalities of text and image.
[0103] S402, using each region in the image as a node, using various relationships between nodes as edges, and constructing an image-text semantic association model through an RBF self-increasing neural network.
[0104] S403, build a learning algorithm on the graph, and effectively spread the text semantic information corresponding to the image level to these image regions, forming a large number of semantic basic unit s...
PUM
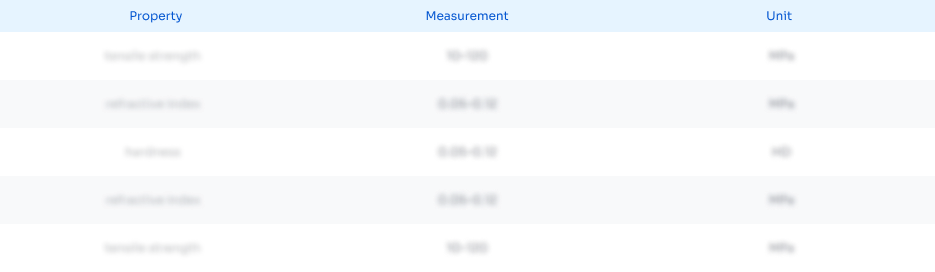
Abstract
Description
Claims
Application Information
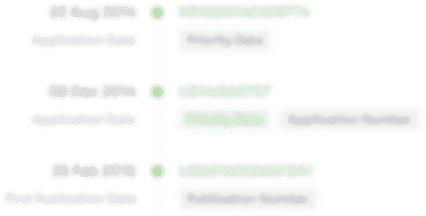
- R&D Engineer
- R&D Manager
- IP Professional
- Industry Leading Data Capabilities
- Powerful AI technology
- Patent DNA Extraction
Browse by: Latest US Patents, China's latest patents, Technical Efficacy Thesaurus, Application Domain, Technology Topic, Popular Technical Reports.
© 2024 PatSnap. All rights reserved.Legal|Privacy policy|Modern Slavery Act Transparency Statement|Sitemap|About US| Contact US: help@patsnap.com