Lightweight fine-grained image recognition method for cross-layer feature interaction in weak supervision scene
A feature interaction and image recognition technology, applied in the field of computer vision, can solve problems such as loss of discriminative information, information loss, and increased running time.
- Summary
- Abstract
- Description
- Claims
- Application Information
AI Technical Summary
Problems solved by technology
Method used
Image
Examples
Embodiment Construction
[0046] Below in conjunction with accompanying drawing and specific embodiment the present invention is described in further detail:
[0047] The invention provides a lightweight fine-grained image recognition method for cross-layer feature interaction in a weakly supervised scene, and solves the technical problem of constructing a fine-grained recognition model using only image-level labels, which reduces the storage of the model while obtaining high recognition accuracy Space and computational cost, making it suitable for large-scale real-world scenarios.
[0048] Such as figure 1 As shown in , a lightweight fine-grained image recognition method based on cross-layer feature interaction in a weakly supervised scene, including the following steps:
[0049] Step 1: In the preprocessing stage, the original image of any size is uniformly scaled to 600×600 pixels, and on this basis, a 448×448 pixel area is cut out with the center of the image as the origin, according to the mean v...
PUM
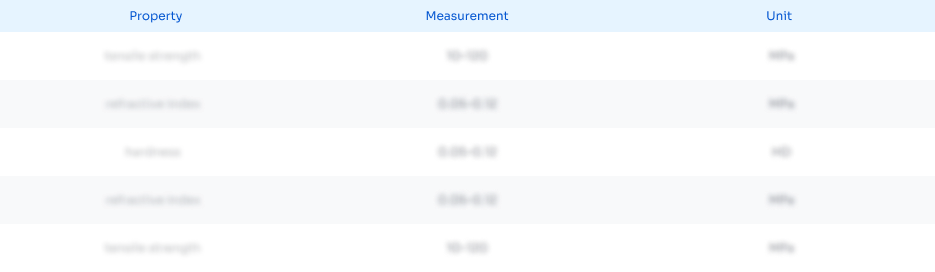
Abstract
Description
Claims
Application Information
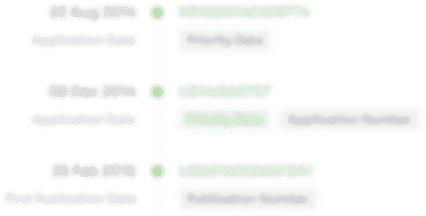
- R&D
- Intellectual Property
- Life Sciences
- Materials
- Tech Scout
- Unparalleled Data Quality
- Higher Quality Content
- 60% Fewer Hallucinations
Browse by: Latest US Patents, China's latest patents, Technical Efficacy Thesaurus, Application Domain, Technology Topic, Popular Technical Reports.
© 2025 PatSnap. All rights reserved.Legal|Privacy policy|Modern Slavery Act Transparency Statement|Sitemap|About US| Contact US: help@patsnap.com