Hyperspectral image classification method for lightweight depth separable convolution feature fusion network
A hyperspectral image and feature fusion technology, applied in the field of hyperspectral image classification, can solve problems such as increasing network depth, and achieve the effects of reducing the amount of network parameters, improving network robustness, and improving classification accuracy
- Summary
- Abstract
- Description
- Claims
- Application Information
AI Technical Summary
Problems solved by technology
Method used
Image
Examples
Embodiment Construction
[0047] The invention provides a hyperspectral image classification method of a lightweight deep separable convolutional feature fusion network, which adopts.
[0048] see figure 1 , a hyperspectral image classification method of a light-weight depth separable convolutional feature fusion network of the present invention, comprising the following steps:
[0049] S1. Data preprocessing;
[0050] S101, processing hyperspectral images
[0051] Due to the large number of bands in the hyperspectral image, the feature information of the spectral dimension is relatively redundant, and the main feature information of the spectral dimension is extracted by PCA dimension reduction.
[0052] S102, normalization processing
[0053] After the original image is dimensionally reduced by PCA, the sample data is normalized; using min-max normalization, that is, the sample data is linearly transformed so that the result is mapped to [0-1]. The conversion formula is as follows:
[0054]
...
PUM
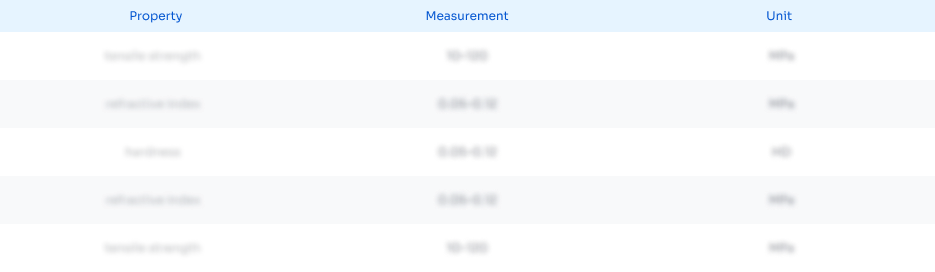
Abstract
Description
Claims
Application Information
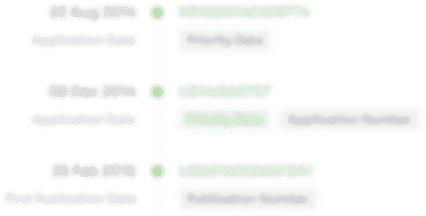
- R&D Engineer
- R&D Manager
- IP Professional
- Industry Leading Data Capabilities
- Powerful AI technology
- Patent DNA Extraction
Browse by: Latest US Patents, China's latest patents, Technical Efficacy Thesaurus, Application Domain, Technology Topic, Popular Technical Reports.
© 2024 PatSnap. All rights reserved.Legal|Privacy policy|Modern Slavery Act Transparency Statement|Sitemap|About US| Contact US: help@patsnap.com