Damaged two-dimensional code recovery method of convolutional auto-encoder in combination with binary segmentation
A convolutional self-encoding and binary segmentation technology, which is applied in the field of damaged two-dimensional code restoration, achieves the effects of sufficient data set types, improved image quality, and strong model generalization ability
- Summary
- Abstract
- Description
- Claims
- Application Information
AI Technical Summary
Problems solved by technology
Method used
Image
Examples
Embodiment 1
[0059] Example 1: see Figure 1 to Figure 2 , A method for recovering damaged two-dimensional codes combined with binary segmentation convolutional autoencoder, including the following steps:
[0060] (1) Prepare the simulated data set and the real data set, together as the training data set, among which,
[0061] Simulation experiment data set: Simulation generates multiple clear QR code images with different data content and styles, and adds different degrees of blur, shading and / or additive noise to them to obtain multiple damaged QR code images;
[0062] Real collection of data sets: use the camera to shoot, keep the relative position of the QR code unchanged, take a clear image of the QR code, then adjust the camera parameters and control its jitter, shoot multiple images with varying degrees of blur and varying degrees of damage Dimension code image;
[0063] Use the clear QR code image as the label corresponding to the damaged QR code image, and the resolution of the damaged QR...
Embodiment 2
[0072] Example 2: see Figure 1 to Figure 5 To better explain the solution of the present invention, on the basis of Example 1, we add the following technical features:
[0073] The binary classification is calculated using the following formula
[0074]
[0075] Where x i Represents the feature representation of the channel i of the output tensor, n represents the two categories of black and white pixels, the value is 2, p(x i ) Is the output probability of the corresponding channel;
[0076] The cross entropy loss function is
[0077]
[0078] Where w(x i ) Is the label of the pixel of the damaged QR code image. The rest is the same as in Example 1.
Embodiment 3
[0079] Example 3: See Figure 1 to Figure 5 In order to better explain the solution of the present invention, on the basis of Example 1, we specifically describe as follows:
[0080] (1) Prepare the simulated data set and the real data set, together as the training data set, among which,
[0081] Simulation experiment data set: The simulation generates multiple clear QR code images with different data content and styles, and adds different degrees of blur, shading and / or additive noise to them to obtain multiple damaged QR code images; The parameter settings of the damaged QR code image are shown in Table 1 below:
[0082] Table 1: Parameter setting range of simulated damaged QR code image
[0083]
[0084] The brightness and dark pixel adjustment ratio parameters of the simulated QR code image are set to 0.8 to 0.9 and 0.1 to 0.2, respectively; the blur radius of the out-of-focus blur is set to 7 to 9; the pixel blur length of the motion blur is set to 18 to 22. Set the blur angle f...
PUM
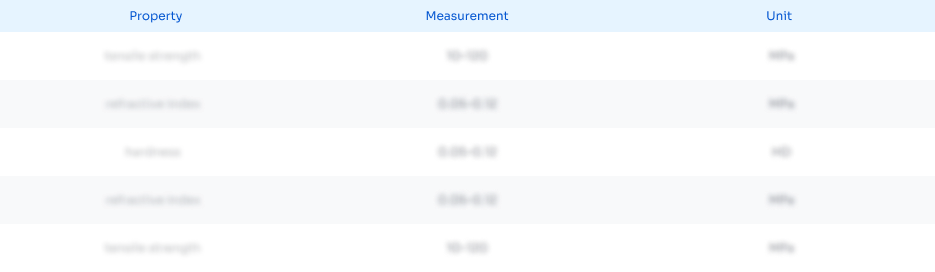
Abstract
Description
Claims
Application Information
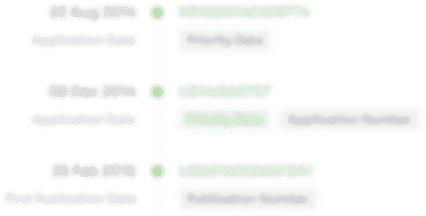
- Generate Ideas
- Intellectual Property
- Life Sciences
- Materials
- Tech Scout
- Unparalleled Data Quality
- Higher Quality Content
- 60% Fewer Hallucinations
Browse by: Latest US Patents, China's latest patents, Technical Efficacy Thesaurus, Application Domain, Technology Topic, Popular Technical Reports.
© 2025 PatSnap. All rights reserved.Legal|Privacy policy|Modern Slavery Act Transparency Statement|Sitemap|About US| Contact US: help@patsnap.com