Semantic feature-based face false detection screening method
A semantic feature, face detection technology, applied in the field of face detection and recognition, can solve problems such as poor application, increased computational complexity, and difficulty in effectively screening falsely detected targets.
- Summary
- Abstract
- Description
- Claims
- Application Information
AI Technical Summary
Problems solved by technology
Method used
Image
Examples
Embodiment 1
[0041] The training data involved in this embodiment mainly includes two parts. One is the face semantic annotation dataset CelebAMask-HQ, which contains a total of 30,000 images with a resolution of 512*512. Annotated 19 categories of semantic segmentation image masks, including all facial components and accessories, namely: skin, nose, left eye, right eye, left eyebrow, right eyebrow, left ear, right ear, mouth, upper lip, lower lip, hair , necks, hats, glasses, earrings, necklaces, clothes and backgrounds. In this embodiment, this data set is mainly used to train the face semantic segmentation model. The other is the face detection result data set FDRFP, which is collected and organized by the inventor independently. It contains a total of 5967 images, including 3231 positive samples and 2736 negative samples, with an image resolution of 112*112. The data set is mainly used for the training of the face misdetection classification integration model in the embodiment after t...
PUM
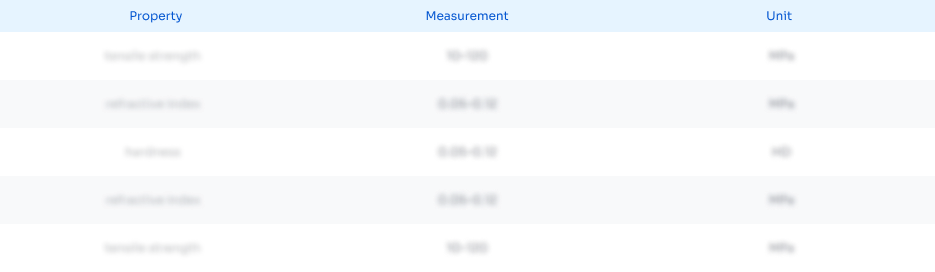
Abstract
Description
Claims
Application Information
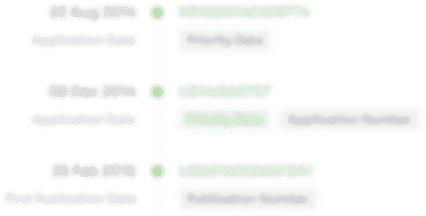
- R&D Engineer
- R&D Manager
- IP Professional
- Industry Leading Data Capabilities
- Powerful AI technology
- Patent DNA Extraction
Browse by: Latest US Patents, China's latest patents, Technical Efficacy Thesaurus, Application Domain, Technology Topic, Popular Technical Reports.
© 2024 PatSnap. All rights reserved.Legal|Privacy policy|Modern Slavery Act Transparency Statement|Sitemap|About US| Contact US: help@patsnap.com