Composite material component defect detection method and device based on generative adversarial learning
A composite material component and defect detection technology, which is applied in image data processing, instrumentation, computing, etc., can solve problems such as difficult convergence of the network, small number of defect samples, and unbalanced categories
- Summary
- Abstract
- Description
- Claims
- Application Information
AI Technical Summary
Problems solved by technology
Method used
Image
Examples
Embodiment 1
[0093] figure 1 It is a schematic flowchart of a defect detection method for composite material components based on generative adversarial learning in an embodiment of the present invention. like figure 1 As shown, the embodiment of the present invention provides a method for detecting defects in composite material components based on generative confrontation learning, the method comprising:
[0094] Step 110: constructing a generative confrontation model of encoding-decoding structure;
[0095] Further, the construction of the generative confrontation model of the encoding-decoding structure includes: adding an encoder E(x, θ e ), where x represents the image to be detected, θ e For network parameter coder E (x) output z=E (x) is the encoding of described image to be detected; Add confrontation network D ' (z, φ), φ is network parameter, adds to the encoding of described image to be detected constraints, so that the encoding z distribution of the image to be detected is c...
Embodiment 2
[0135] Based on the same inventive concept as the method for detecting defects in composite material components based on generative confrontation learning in the foregoing embodiments, the present invention also provides a device for detecting defects in composite material components based on generative confrontation learning, such as figure 2 As shown, the device includes:
[0136] The first construction unit 11, the first construction unit 11 is used to construct the generated confrontation model of the encoding-decoding structure;
[0137] A first obtaining unit 12, the first obtaining unit 12 is used to obtain a defect data set according to the image to be detected
[0138] The first training unit 13, the first training unit 13 is used to utilize the defect data set training the generative adversarial model;
[0139] A second obtaining unit 14, the second obtaining unit 14 is used to screen and obtain suspected defects according to the trained generation confrontatio...
Embodiment 3
[0174] Based on the same inventive concept as the method for detecting defects of composite material components based on generative confrontation learning in the foregoing embodiments, the present invention also provides a device for detecting defects of composite material components based on generative confrontation learning, on which a computer program is stored. When the program is executed by the processor, the steps of any one of the above-mentioned methods for detecting defects in composite material components based on generative adversarial learning are realized.
[0175] Among them, in image 3 In, bus architecture (represented by bus 300), bus 300 may include any number of interconnected buses and bridges, bus 300 will include one or more processors represented by processor 302 and various types of memory represented by memory 304 circuits linked together. The bus 300 may also link together various other circuits, such as peripherals, voltage regulators, and power ma...
PUM
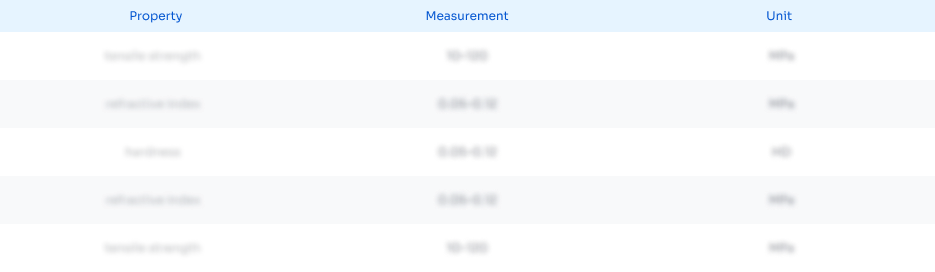
Abstract
Description
Claims
Application Information
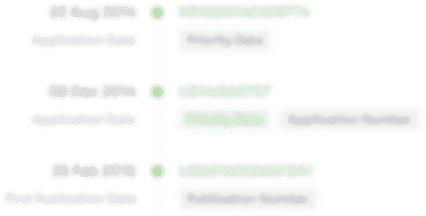
- R&D Engineer
- R&D Manager
- IP Professional
- Industry Leading Data Capabilities
- Powerful AI technology
- Patent DNA Extraction
Browse by: Latest US Patents, China's latest patents, Technical Efficacy Thesaurus, Application Domain, Technology Topic, Popular Technical Reports.
© 2024 PatSnap. All rights reserved.Legal|Privacy policy|Modern Slavery Act Transparency Statement|Sitemap|About US| Contact US: help@patsnap.com