Sample processing method and system based on kernel extreme learning machine
A technology of nuclear extreme learning machine and processing method, which is applied in the field of soft sensor modeling and application in industrial production process, which can solve the problems of poor stability of soft sensor model and high operation cost, so as to realize soft sensor, reduce operation cost and reduce manual marking cost effect
- Summary
- Abstract
- Description
- Claims
- Application Information
AI Technical Summary
Problems solved by technology
Method used
Image
Examples
specific Embodiment
[0130] The method proposed by the present invention is applied to the soft measurement of the sulfur recovery process (SRU, Sulfur Recovery Unit). The SRU collects 2000 sets of data in total, from which 1000 sets of data are selected as a training set, and 1000 sets of data are used as a test set. Initially marked in the training set There are 10 samples, 990 unlabeled samples, and the labeling rate is 1%. The KELM method is used to train the model in the iterative process.
[0131] First, the experimental analysis is carried out on the learning step size of the iterative update process (the number of labeled samples per iteration). As the learning step size num increases, more labeled samples are added to the training set to optimize the KELM model, and the model performance is improved. faster, but the cost of manual labeling also increases. Further, in the case of the same number of labeled samples, the simulation experiment is re-run. The smaller the learning step size in ...
PUM
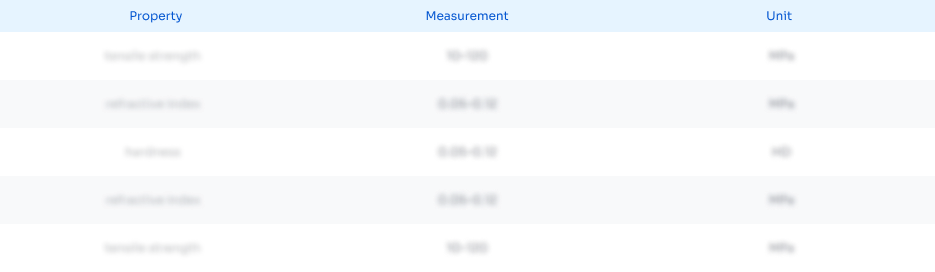
Abstract
Description
Claims
Application Information
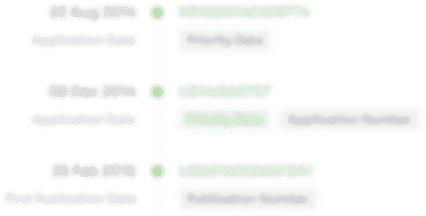
- R&D
- Intellectual Property
- Life Sciences
- Materials
- Tech Scout
- Unparalleled Data Quality
- Higher Quality Content
- 60% Fewer Hallucinations
Browse by: Latest US Patents, China's latest patents, Technical Efficacy Thesaurus, Application Domain, Technology Topic, Popular Technical Reports.
© 2025 PatSnap. All rights reserved.Legal|Privacy policy|Modern Slavery Act Transparency Statement|Sitemap|About US| Contact US: help@patsnap.com