Underexposure image recovery method based on deep learning
A technology of image restoration and deep learning, applied in the direction of neural learning methods, image enhancement, image analysis, etc., can solve the problems that the image cannot be guaranteed at the same time, the detailed information of the area is lost, and the image is prone to whitening areas, etc., to achieve clear details and less noise , improve the effect of optimization
- Summary
- Abstract
- Description
- Claims
- Application Information
AI Technical Summary
Problems solved by technology
Method used
Image
Examples
Embodiment Construction
[0032] In order to make the objects, features and advantages of the present invention more comprehensible, specific implementations of the present invention will be described in detail below in conjunction with the accompanying drawings.
[0033] In order to better understand the image restoration method of the present invention, the image restoration network of the present invention will be introduced in detail below.
[0034] 1. The specific implementation of underexposed image restoration network
[0035] Such as figure 1 As shown, the image enhancement process is divided into three steps: decomposition, adjustment and reconstruction. In the decomposition step, the Multiscale-Decom-Net based on the encoder-decoder structure outputs the input original image as feature maps of different resolutions. , taking the structure in the figure as an example, the original image is transformed into feature maps of three resolutions after being processed by the Multiscale-Decom-Net net...
PUM
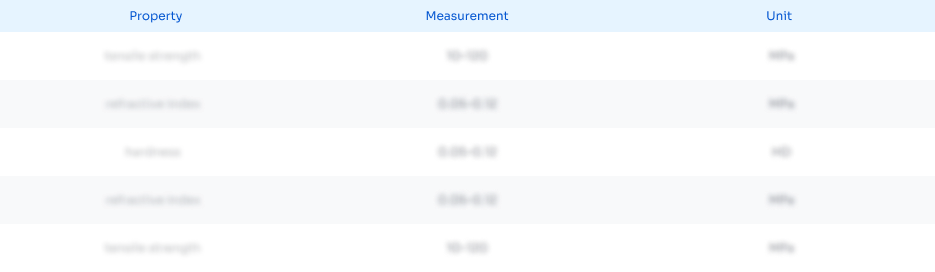
Abstract
Description
Claims
Application Information
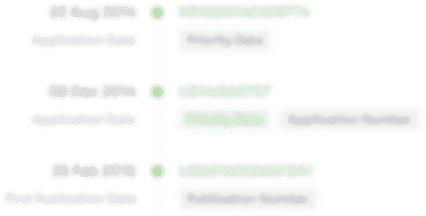
- R&D Engineer
- R&D Manager
- IP Professional
- Industry Leading Data Capabilities
- Powerful AI technology
- Patent DNA Extraction
Browse by: Latest US Patents, China's latest patents, Technical Efficacy Thesaurus, Application Domain, Technology Topic, Popular Technical Reports.
© 2024 PatSnap. All rights reserved.Legal|Privacy policy|Modern Slavery Act Transparency Statement|Sitemap|About US| Contact US: help@patsnap.com