Traffic flow prediction method and system for urban road network
A technology of urban road network and traffic flow, applied in traffic flow detection, traffic control system of road vehicles, traffic control system, etc., can solve problems such as inability to accurately predict traffic flow and lack of flexibility.
- Summary
- Abstract
- Description
- Claims
- Application Information
AI Technical Summary
Problems solved by technology
Method used
Image
Examples
Embodiment 1
[0057] figure 1 It is a flow chart of Embodiment 1 of the traffic flow prediction method for urban road network of the present invention. see figure 1 , the traffic flow prediction methods of the urban road network include:
[0058] Step 101: establish a training data set; the training data set includes an adjacency matrix and a time-series vector matrix; the adjacency matrix indicates whether each road section in the urban road network is adjacent; the time-series vector matrix indicates that each road section used to be Traffic flow for a time period; each of said time periods has the same time interval.
[0059] This step 101 specifically includes:
[0060] The urban road network is modeled to obtain an undirected graph of the urban road network; the undirected graph represents the topological structure of the urban road network; the nodes of the undirected graph represent road sections, and the edges of the undirected graph represent road sections connected.
[0061] ...
Embodiment 2
[0076] figure 2 It is an overview schematic diagram of Embodiment 2 of the traffic flow prediction method for urban road network of the present invention. see figure 2 , the traffic flow prediction method of the urban road network is based on the graph wavelet attention gating recurrent neural network model to predict the traffic flow of the urban road network, such as figure 2 Method overview:
[0077] First, the urban road network is modeled as an undirected graph structure. The nodes of the graph represent road segments, and the edges of the graph represent the connectivity between intersections. The time series of road segments is the attribute feature of the traffic flow of nodes. The time series of a road segment is the traffic flow of a certain road segment within a time period of 5 minutes.
[0078] Second, using the above-mentioned graph and historical traffic time series as input, the graph wavelet attention-gated recurrent neural network model is used to captu...
Embodiment 3
[0129] Figure 6 It is a structural diagram of Embodiment 3 of the traffic flow forecasting system of the urban road network of the present invention. see Figure 6 , the traffic flow prediction system of the urban road network includes:
[0130] The training data set building module 601 is used to set up the training data set; the training data set includes an adjacency matrix and a time series vector matrix; the adjacency matrix indicates whether each road section in the urban road network is adjacent; the time series vector The matrix represents the traffic flow of each road segment for each time period in the past; each said time period has the same time interval.
[0131] In the training data set establishing module 601, the time interval is 5 minutes.
[0132] The training data set building module 601 specifically includes:
[0133] The urban road network modeling unit is used to model the urban road network to obtain an undirected graph of the urban road network; th...
PUM
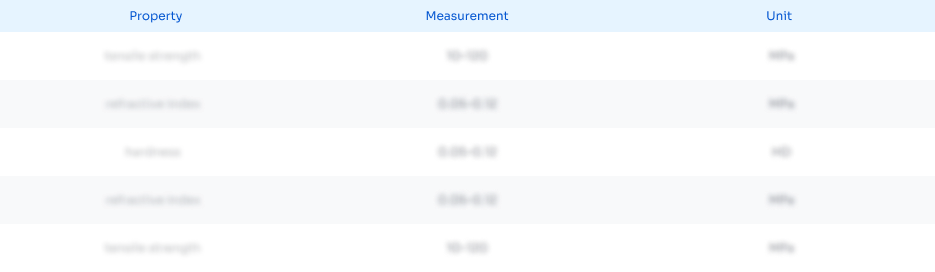
Abstract
Description
Claims
Application Information
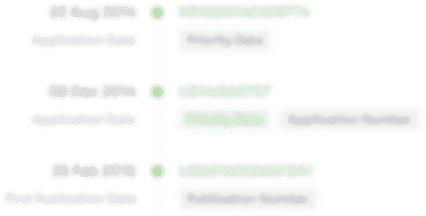
- R&D Engineer
- R&D Manager
- IP Professional
- Industry Leading Data Capabilities
- Powerful AI technology
- Patent DNA Extraction
Browse by: Latest US Patents, China's latest patents, Technical Efficacy Thesaurus, Application Domain, Technology Topic, Popular Technical Reports.
© 2024 PatSnap. All rights reserved.Legal|Privacy policy|Modern Slavery Act Transparency Statement|Sitemap|About US| Contact US: help@patsnap.com