Hyperspectral image classification method based on auto-encoder and 3D deep residual network
A hyperspectral image and autoencoder technology, which is applied in the field of hyperspectral image classification based on autoencoder and 3D deep residual network, and can solve problems such as classification of difficult remote sensing hyperspectral images.
- Summary
- Abstract
- Description
- Claims
- Application Information
AI Technical Summary
Problems solved by technology
Method used
Image
Examples
Embodiment 1
[0174] Embodiment 1: In order to verify the impact of different parameter settings on the classification accuracy in the method proposed by the present invention, several factors that affect the classification effect of the model in the SAE-3DDRN method will be analyzed, namely, the dimensionality reduction d of the self-encoding network and the input sample size Size w, residual network structure, learning rate size lr. For the convenience of description, this section will randomly select 10% of the training samples from each type of ground object in the Indian Pines dataset, and the rest will be used as test samples, and the average of the classification accuracy of 10 experiments will be used as the experimental result.
[0175] For high-dimensional hyperspectral remote sensing images, there is a large amount of redundant information. If the input data dimension is too large, the complexity of the model will be increased. Therefore, reducing the dimension to the appropriate ...
Embodiment 2
[0179] Embodiment 2: In order to further verify the validity of the method proposed by the present invention, the PaviaUniversity and Indian Pines data sets will be used for verification below, and the classification method SAE-3DDRN described in the present invention will be combined with some traditional hyperspectral classification methods Compared with the current mainstream algorithms, the number of training samples used by each classification method is exactly the same.
[0180] Table 5 Classification accuracy of different classification methods on the Indian Pines dataset
[0181]
[0182]
[0183] right image 3 The hyperspectral image of Indian Pines shown is classified, and 10% samples are randomly selected from each type of ground object as the training set, and the remaining samples are used as the test set. On this data set, the classification method SAE-3DDRN proposed by the present invention uses a 5-layer stacked autoencoder structure to reduce the dimen...
Embodiment 3
[0187] Embodiment 3: In order to verify the impact of the classification method of the present invention on the hyperspectral classification accuracy under different sample ratios, this paper sets four training sample ratios of 5%, 10%, 15%, and 20%, respectively from Indian Pines and PaviaUniversity data sets are randomly selected, and the average and standard deviation of ten classification results are taken for each experimental result. Figure 10 , Figure 11 They are the point-line diagrams of OA, AA, and Kapp a changes under different training sample ratios of the method SAE-3DDRN described in this paper under the Indian Pines and Pavia University datasets. From Figure 10 , Figure 11 It can be seen that the SAE-3DDRN method proposed in this paper shows the best classification accuracy in OA, AA, and Kappa under different proportion samples of the two datasets. As the training sample size increases, the classification accuracy of most classification methods continues...
PUM
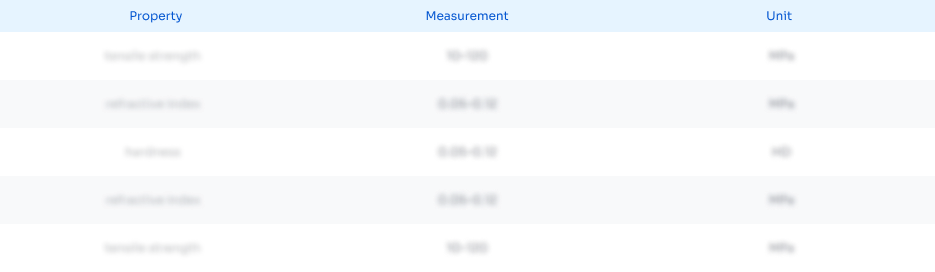
Abstract
Description
Claims
Application Information
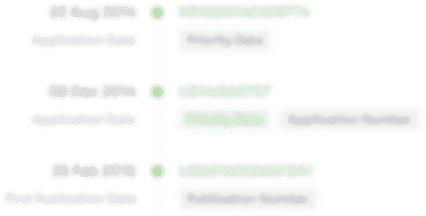
- R&D Engineer
- R&D Manager
- IP Professional
- Industry Leading Data Capabilities
- Powerful AI technology
- Patent DNA Extraction
Browse by: Latest US Patents, China's latest patents, Technical Efficacy Thesaurus, Application Domain, Technology Topic, Popular Technical Reports.
© 2024 PatSnap. All rights reserved.Legal|Privacy policy|Modern Slavery Act Transparency Statement|Sitemap|About US| Contact US: help@patsnap.com