Saliency detection method for cross-modal enhancement and improvement of loss function
A technology of loss function and detection method, which is applied in the field of saliency detection of deep learning, can solve the problems that the results cannot reach the optimal effect, the prediction results are rough, and the detection accuracy is low.
- Summary
- Abstract
- Description
- Claims
- Application Information
AI Technical Summary
Problems solved by technology
Method used
Image
Examples
Embodiment Construction
[0053] The present invention will be further described in detail below in conjunction with the accompanying drawings and embodiments.
[0054] The present invention proposes a saliency detection method for cross-modal enhancement and improved loss function, which includes two processes of a training phase and a testing phase.
[0055] The specific steps of the described training phase process are:
[0056] Step 1_1: Select Q original color images, the depth images corresponding to each original color image and the corresponding true salient detection images, and form a training set, and record the qth original color image in the training set as focus training on The corresponding depth image is recorded as focus training on The corresponding ground truth salient detection image is denoted as Similarly, select Q' original color images, the depth images corresponding to each original color image, and the corresponding real salient detection images to form a verification...
PUM
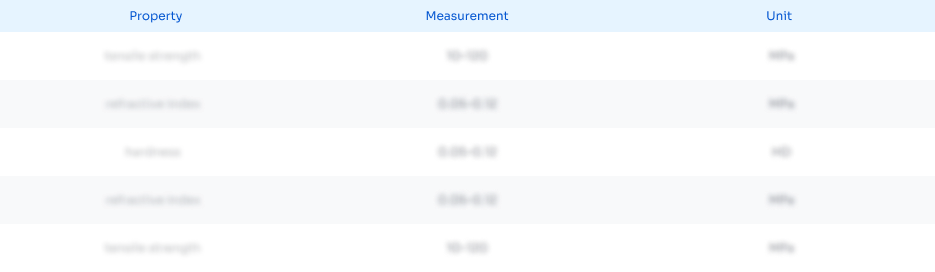
Abstract
Description
Claims
Application Information
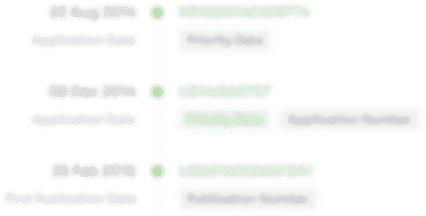
- R&D Engineer
- R&D Manager
- IP Professional
- Industry Leading Data Capabilities
- Powerful AI technology
- Patent DNA Extraction
Browse by: Latest US Patents, China's latest patents, Technical Efficacy Thesaurus, Application Domain, Technology Topic, Popular Technical Reports.
© 2024 PatSnap. All rights reserved.Legal|Privacy policy|Modern Slavery Act Transparency Statement|Sitemap|About US| Contact US: help@patsnap.com