Mobile edge computing offload method based on multi-agent reinforcement learning
A technology of reinforcement learning and edge computing, applied in the field of edge computing and wireless network, it can solve the problems of long computing time overhead, difficulty and difficulty in adaptively tracking the dynamic environment of the network, and achieve the effect of optimal overall network utility.
- Summary
- Abstract
- Description
- Claims
- Application Information
AI Technical Summary
Problems solved by technology
Method used
Image
Examples
Embodiment Construction
[0073] Taking the mobile edge system composed of 4 user equipments and 2 base stations as an example, it is assumed that there are 2 channels available between each user and the base station, each channel bandwidth is 0.6MHz, and the channel gain obeys the Rayleigh distribution. The length of each time slot is 1 second, assuming that the energy collected by the user through wireless charging in each time slot obeys the Poisson distribution. The maximum CPU cycle frequencies of the two base stations are 10GHz and 30GHz, respectively, and the CPU cycle frequencies assigned to each task are 5GHz and 10GHz, respectively. The data size of tasks generated by each device at the beginning of each time slot and the CPU cycles to be consumed are randomly generated within a certain range.
[0074] The following table shows the specific program flow based on the multi-agent reinforcement learning algorithm:
[0075]
[0076]
[0077] The online and target neural networks of Actor a...
PUM
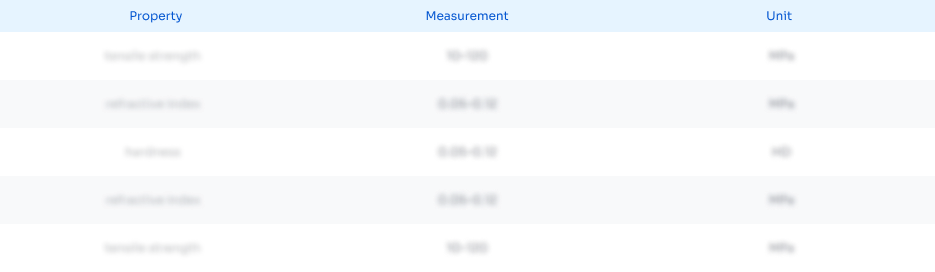
Abstract
Description
Claims
Application Information
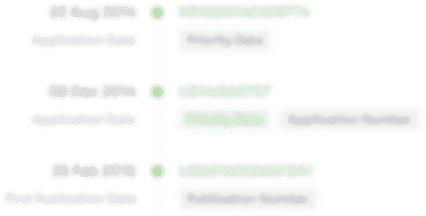
- R&D
- Intellectual Property
- Life Sciences
- Materials
- Tech Scout
- Unparalleled Data Quality
- Higher Quality Content
- 60% Fewer Hallucinations
Browse by: Latest US Patents, China's latest patents, Technical Efficacy Thesaurus, Application Domain, Technology Topic, Popular Technical Reports.
© 2025 PatSnap. All rights reserved.Legal|Privacy policy|Modern Slavery Act Transparency Statement|Sitemap|About US| Contact US: help@patsnap.com