Short-term photovoltaic output probability prediction method based on simplest gated neural network
A neural network and probability prediction technology, applied in the field of short-term photovoltaic output probability prediction, can solve the problems of LSTM difficult to successfully converge, low prediction accuracy, weak generalization ability, etc., and achieve the effect of rapid construction, improved accuracy, and high coverage
- Summary
- Abstract
- Description
- Claims
- Application Information
AI Technical Summary
Problems solved by technology
Method used
Image
Examples
Embodiment
[0046] The present invention proposes a photovoltaic output probability prediction method based on maximum information coefficient (MIC) correlation analysis and improved LSTM neural network, and provides a hybrid framework combining quantile regression and arbitrary point prediction methods, which can predict photovoltaic output , and quantify the uncertainty of prediction. In order to add time information to the model and further improve the prediction accuracy, a hybrid model combining quantile regression and improved LSTM is proposed for the distributed photovoltaic ultra-short-term probability prediction method: choose After appropriate input features, considering the high dimensionality of weather features in the input features and weak correlation with the output, MIC is used for correlation analysis of the photovoltaic output impact sequence, and the input features with the highest correlation with photovoltaic output are screened out. Improve the data density while ret...
PUM
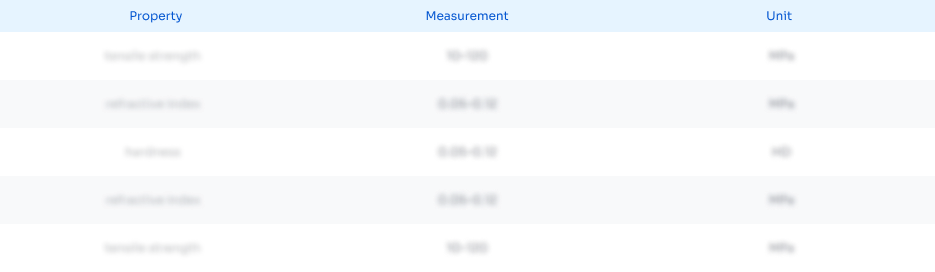
Abstract
Description
Claims
Application Information
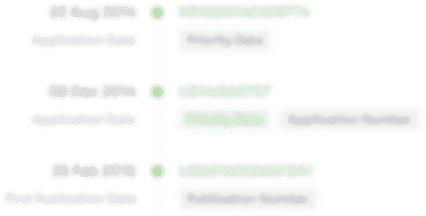
- R&D Engineer
- R&D Manager
- IP Professional
- Industry Leading Data Capabilities
- Powerful AI technology
- Patent DNA Extraction
Browse by: Latest US Patents, China's latest patents, Technical Efficacy Thesaurus, Application Domain, Technology Topic, Popular Technical Reports.
© 2024 PatSnap. All rights reserved.Legal|Privacy policy|Modern Slavery Act Transparency Statement|Sitemap|About US| Contact US: help@patsnap.com