Medical image multi-classification recognition system based on improved ResNet
A recognition system and medical image technology, applied in the field of medical image processing, can solve problems such as low cell classification accuracy, unsatisfactory network model classification and recognition effect, and unbalanced number of cell samples, so as to improve recognition accuracy and reduce background interference , the effect of reducing the amount of calculation
- Summary
- Abstract
- Description
- Claims
- Application Information
AI Technical Summary
Problems solved by technology
Method used
Image
Examples
Embodiment Construction
[0039] The present invention is based on the improved ResNet medical image multi-classification recognition system, including two modules: a positioning module and a classification module, wherein:
[0040] The positioning module uses the Hourglass network model to extract the features of the input granulocyte picture, and locates all the cells in the granulocyte picture separately. The positioning of the cells is realized by extracting the center point of the target cell, and then the positioned cells are cut out. Come out, leaving only a single complete cell in the field of view, and normalize the size of all the cropped cells;
[0041] Such as figure 1 As shown, the Hourglass network module is a symmetrical structure, including a four-layer lower convolutional layer group and a four-layer upper convolutional layer group; and cross-layer connections are performed through feature map fusion in the symmetrical upper and lower convolutional layer groups:
[0042] The first low...
PUM
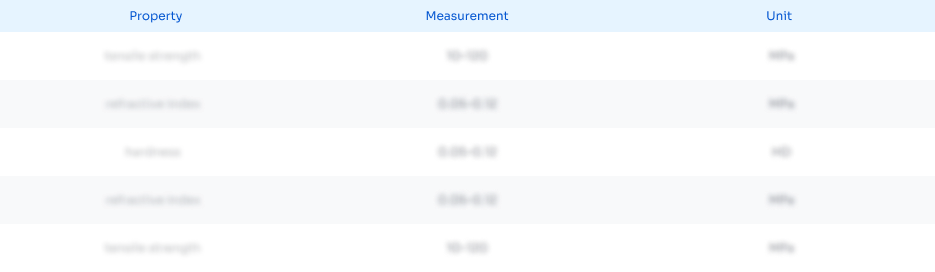
Abstract
Description
Claims
Application Information
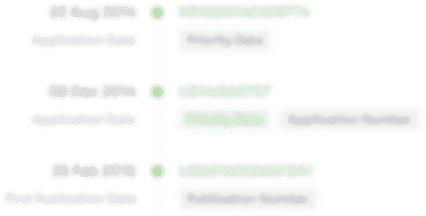
- R&D Engineer
- R&D Manager
- IP Professional
- Industry Leading Data Capabilities
- Powerful AI technology
- Patent DNA Extraction
Browse by: Latest US Patents, China's latest patents, Technical Efficacy Thesaurus, Application Domain, Technology Topic, Popular Technical Reports.
© 2024 PatSnap. All rights reserved.Legal|Privacy policy|Modern Slavery Act Transparency Statement|Sitemap|About US| Contact US: help@patsnap.com