Robust communication radiation source intelligent identification method based on deep learning
A deep learning and intelligent recognition technology, applied in the field of machine learning, can solve the problems of discounted recognition accuracy and poor robustness, and achieve the effects of strong adaptability, improved matching degree, and high recognition sensitivity
- Summary
- Abstract
- Description
- Claims
- Application Information
AI Technical Summary
Problems solved by technology
Method used
Image
Examples
Embodiment 1
[0030]This example discloses a method of intelligent recognition based on deep learning robust communication radiation source, including:
[0031]A. Data pretreatment
[0032]Such asfigure 1 As shown, the signal preprocessing phase passes through the carrier frequency estimation, the lower frequency conversion, phase calculation, slimming, data standardization, and the like, the original data processing is a baseband signal data conducive to the extraction of radiation source subtle features in deep neural network.
[0033]A1. Bundry Estimation
[0034]Since there is an unknown frequency offset, the carrier frequency of the signal is first to be estimated before the intermediate frequency signal is downconverted. For QPSK signals, the four spectrums also have a strong discrete spectrum component in the quad-fold frequency, which is more powerful, and the carrier frequency estimation is simple, and the amount of operation is small based on the four-fold frequency. Higher, the carrier frequency o...
Embodiment 2
[0096]This example discloses a method of intelligent recognition based on deep learning robust communication radiation source, including:
[0097]The pretreatment step of signal sample data. The pre-processing step is cleaned on the signal sample data to make the sample data easy to train the learning model. The pre-processing step includes the process of carrier frequency estimation, down frequency, phase compensation, mining sample, and data standardization processing.
[0098]After pretreatment, the signal sample data is enhanced by using the signal-to-noise ratio evaluating the test set assessed in the test environment. The so-called test environment is an application environment, the test set is also the signal sample collected in the application environment, in this regard as the data enhancement reference to the training sample, making training samples to be more adapted to the actual environment.
[0099]Based on the design of the design model architecture, the CNN module is utilized...
Embodiment 3
[0103]The present embodiment will be described in accordance with the data acquired by the actual device, and the identification process will be described. Since the data used is collected by the actual equipment, there is a certain noise, frequency offset, and meta.
[0104]This embodiment contains 30 signal samples generated by 30 communication radiation sources. The signal is 70 MHz intermediate frequency signal sampling, the sample rate is 30 mSPS, the signal carrier frequency is 1000 MHz, the modulation method is QPSK, the modulation rate is 1M baud / s, the test data The blind selection module is an unknown radiation source signal that is not included in the training data.
[0105]First, pretreatment of the collected data signals, such asfigure 1 As shown, the specific training steps of the depth learning network are as follows:
[0106]Step 1, the carrier frequency estimation
[0107]For QPSK signals, there will be a strong discrete spectrum component in tetracy, and the carrier frequenc...
PUM
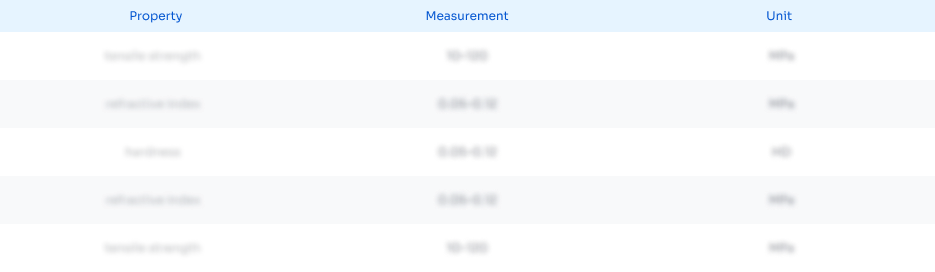
Abstract
Description
Claims
Application Information
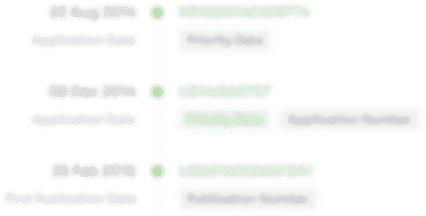
- R&D Engineer
- R&D Manager
- IP Professional
- Industry Leading Data Capabilities
- Powerful AI technology
- Patent DNA Extraction
Browse by: Latest US Patents, China's latest patents, Technical Efficacy Thesaurus, Application Domain, Technology Topic, Popular Technical Reports.
© 2024 PatSnap. All rights reserved.Legal|Privacy policy|Modern Slavery Act Transparency Statement|Sitemap|About US| Contact US: help@patsnap.com