Hyperspectral image classification method based on self-paced learning double-flow multi-scale dense connection network
A hyperspectral image and densely connected technology, which is applied in the field of hyperspectral image classification based on self-paced learning two-stream multi-scale densely connected network, can solve the problems that the spatial resolution of image blocks should not be too large, time-consuming, and huge training data. Achieve the effect of reducing spectral feature redundancy, improving extraction effect, and removing spectral redundancy
- Summary
- Abstract
- Description
- Claims
- Application Information
AI Technical Summary
Problems solved by technology
Method used
Image
Examples
Embodiment
[0095] Such as figure 1 As shown, the specific implementation steps of the hyperspectral image classification method based on the self-paced learning dual-stream multi-scale densely connected network of the present invention are as follows:
[0096] 1a. Read in the hyperspectral image X with dimensions W×H×C 0 , where W, H, and C represent the width, height, and spectral dimension of the hyperspectral image, respectively, and there are K categories of pixels in total.
[0097] Perform unsupervised band selection and dimensionality reduction on the hyperspectral image to obtain C2 important band images, and the hyperspectral image after dimensionality reduction X 1 The dimension is W×H×C2.
[0098] Let D KL (i, j) is the K-L divergence of the j-th band of the hyperspectral image relative to the i-th band, O(i, j) is the Euclidean distance between the j-th band of the hyperspectral image and the i-th band image matrix, A(i,j) is the comprehensive difference value, the formul...
PUM
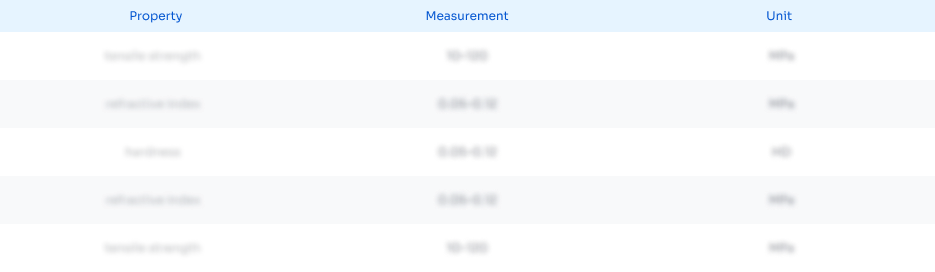
Abstract
Description
Claims
Application Information
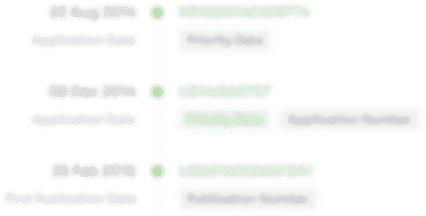
- R&D Engineer
- R&D Manager
- IP Professional
- Industry Leading Data Capabilities
- Powerful AI technology
- Patent DNA Extraction
Browse by: Latest US Patents, China's latest patents, Technical Efficacy Thesaurus, Application Domain, Technology Topic, Popular Technical Reports.
© 2024 PatSnap. All rights reserved.Legal|Privacy policy|Modern Slavery Act Transparency Statement|Sitemap|About US| Contact US: help@patsnap.com