A multi-modal security monitoring method based on deep learning image processing
An image processing and deep learning technology, applied in neural learning methods, genetic models, character and pattern recognition, etc., can solve the problems of high equipment requirements, increased cost and overhead, and poor applicability, and achieve high tracking monitoring, The effect of increasing the detection blind area and enriching the amount of data
- Summary
- Abstract
- Description
- Claims
- Application Information
AI Technical Summary
Problems solved by technology
Method used
Image
Examples
Embodiment 1
[0046] This embodiment proposes a multi-mode security monitoring method based on deep learning image processing, such as figure 1 , figure 2 shown, including the following steps.
[0047] Step 1: Build a global map model; specifically, build a global map model of the monitored site.
[0048] Step 2: Divide sub-areas and install surveillance cameras; specifically, divide multiple different security monitoring sub-areas under the constructed global map model, divide different security monitoring sub-areas into different monitoring levels, and monitor each security monitoring sub-area. Corresponding surveillance cameras are installed in the monitoring sub-area and run for a period of time.
[0049] Step 3: Prepare the pre-training set; specifically, select the image database as the pre-training set for pre-training, obtain the pre-training model, and retrieve all the monitoring images of the historical surveillance cameras in the security monitoring sub-area for enriching the ...
Embodiment 2
[0062] In this embodiment, on the basis of the above-mentioned embodiment 1, in order to better realize the present invention, further, in the step 4, the specific operation of sorting in the monitoring display queue according to the high and low intervals of the monitoring intensity level is: set the first queue and the second queue, first add the two surveillance cameras with the highest monitoring intensity level to the first queue and the second queue respectively, and then add the two surveillance cameras with the lowest monitoring intensity level to the first queue and the second queue respectively Then add the two surveillance cameras with the highest monitoring intensity level among the unsorted surveillance cameras to the third place in the first queue and the second queue respectively, and then add the two surveillance cameras with the lowest monitoring intensity level among the unsorted surveillance cameras Surveillance cameras are respectively added to the fourth of...
Embodiment 3
[0066] In this embodiment, on the basis of any one of the above-mentioned embodiments 1-2, in order to better realize the present invention, further, in the step 4, the preloading time D is set, when a certain monitoring camera is on the monitoring screen When there is still time D left in the display duration of the monitoring sequence, the images collected by the surveillance cameras ranked last in the monitoring sorting queue will be preloaded. When the display duration of a surveillance camera on the surveillance screen is exhausted, the preloaded images will be used for Replace display.
[0067] Working principle: as Figure 5 As shown in , assuming that three surveillance cameras in the detection display queue are used for display at one time, in Figure 5 in the first Figure 4 No. 1, No. 11, and No. 3 surveillance cameras in the detection display queue are displayed, and then when there is time D left for the display time of one of the surveillance cameras No. 1, 11,...
PUM
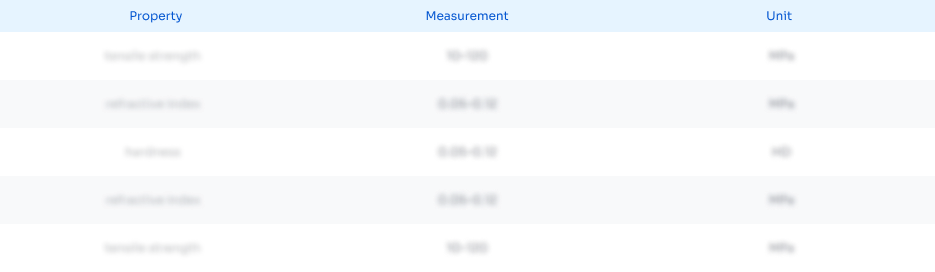
Abstract
Description
Claims
Application Information
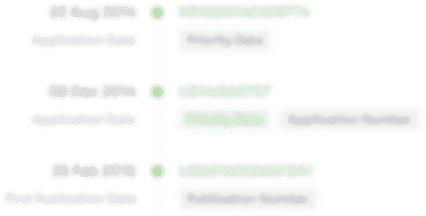
- R&D
- Intellectual Property
- Life Sciences
- Materials
- Tech Scout
- Unparalleled Data Quality
- Higher Quality Content
- 60% Fewer Hallucinations
Browse by: Latest US Patents, China's latest patents, Technical Efficacy Thesaurus, Application Domain, Technology Topic, Popular Technical Reports.
© 2025 PatSnap. All rights reserved.Legal|Privacy policy|Modern Slavery Act Transparency Statement|Sitemap|About US| Contact US: help@patsnap.com