Aurora motion characterization method based on unsupervised deep optical flow network
An unsupervised, aurora technology, applied in the field of video analysis, which can solve the problems of difficulty in manually labeling pixel-level aurora optical flow fields, inability to manually estimate aurora motion methods, and inability to obtain accurate aurora optical flow fields.
- Summary
- Abstract
- Description
- Claims
- Application Information
AI Technical Summary
Problems solved by technology
Method used
Image
Examples
Embodiment Construction
[0048] Below in conjunction with accompanying drawing, implementation steps and technical effects of the present invention are described in further detail:
[0049] A method for characterization of aurora motion based on an unsupervised deep optical flow network, comprising the following steps:
[0050] Step 1: Extract each frame of the original aurora image in the aurora observation video, preprocess each frame of the original aurora image, sort the preprocessed aurora images by time, and obtain a continuous all-sky aurora image sequence;
[0051] Step 2: The optical flow network is trained using a continuous sequence of all-sky aurora images.
[0052] 2.1) Input two consecutive frames of all-sky aurora image I 1 and I 2 , using the same feature pyramid extraction network to extract the feature maps of the two images respectively. The first-level feature map of the feature pyramid extraction network is the input image, and the number of channels is 3; the number of channel...
PUM
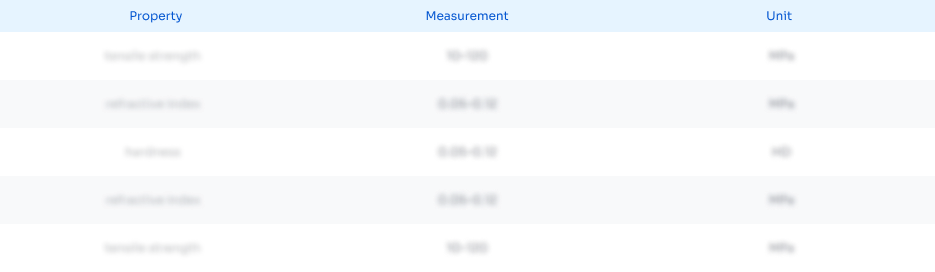
Abstract
Description
Claims
Application Information
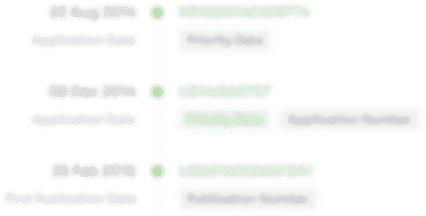
- R&D Engineer
- R&D Manager
- IP Professional
- Industry Leading Data Capabilities
- Powerful AI technology
- Patent DNA Extraction
Browse by: Latest US Patents, China's latest patents, Technical Efficacy Thesaurus, Application Domain, Technology Topic, Popular Technical Reports.
© 2024 PatSnap. All rights reserved.Legal|Privacy policy|Modern Slavery Act Transparency Statement|Sitemap|About US| Contact US: help@patsnap.com