High-generalization cross-domain road scene semantic segmentation method and system
A semantic segmentation and generalization technology, applied in the field of cross-domain road scene semantic segmentation methods and systems, can solve the problems of poor generalization performance and high target domain data dependence
- Summary
- Abstract
- Description
- Claims
- Application Information
AI Technical Summary
Problems solved by technology
Method used
Image
Examples
Embodiment Construction
[0056] In order to facilitate the understanding of those skilled in the art, the present invention will be further described below in conjunction with the embodiments and accompanying drawings, and the contents mentioned in the embodiments are not intended to limit the present invention.
[0057] In the current semantic segmentation method, it is difficult to obtain the image data of the target domain in advance. Taking autonomous driving as an example, it is impossible for the operator to obtain the road images of all target areas in advance, and the existing segmentation methods can only be used for known target domains. Segmentation, so the trained model can only be applied to this specific domain and cannot be generalized to other target domains.
[0058] figure 1A flowchart showing a highly generalized cross-domain road scene semantic segmentation method, including:
[0059] S10: Generate a virtual image and a corresponding label through a game engine.
[0060] Since th...
PUM
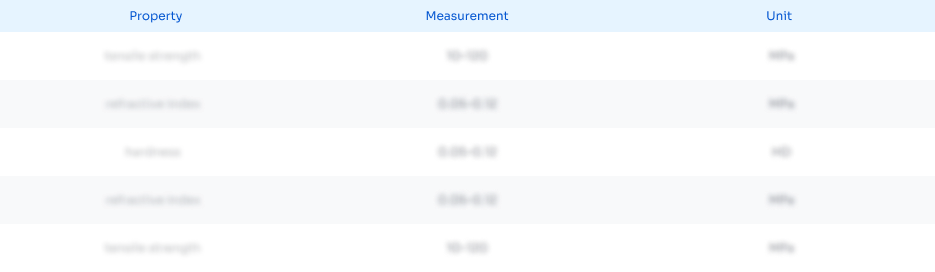
Abstract
Description
Claims
Application Information
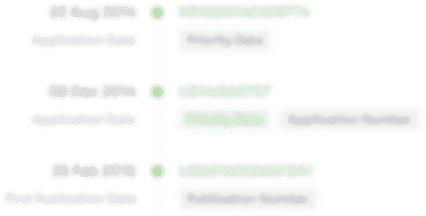
- R&D Engineer
- R&D Manager
- IP Professional
- Industry Leading Data Capabilities
- Powerful AI technology
- Patent DNA Extraction
Browse by: Latest US Patents, China's latest patents, Technical Efficacy Thesaurus, Application Domain, Technology Topic, Popular Technical Reports.
© 2024 PatSnap. All rights reserved.Legal|Privacy policy|Modern Slavery Act Transparency Statement|Sitemap|About US| Contact US: help@patsnap.com