CNN classification model and generative adversarial network-based motor imagery classification method and system
A technology of motion imagery and classification models, applied in neural learning methods, biological neural network models, character and pattern recognition, etc., can solve problems such as incomplete solutions, and achieve the effect of improving quality
- Summary
- Abstract
- Description
- Claims
- Application Information
AI Technical Summary
Problems solved by technology
Method used
Image
Examples
Embodiment 1
[0061] Such as figure 1 , this embodiment proposes a motion imagery classification method based on a CNN classification model and a generative confrontation network, including steps S1 to S3:
[0062] S1. Data preprocessing: Eliminate part of the noise through ICA on the collected MI raw data, eliminate unimportant or interfering signals through a band-pass filter, and perform feature extraction through wavelet transform and fast Fourier transform;
[0063] S2. Data expansion: Generate high-quality MI data through long-term short-term memory network and generative confrontation network;
[0064] S3. Data classification: improve classification performance and obtain classification results through multi-output convolutional neural network;
[0065] Wherein, the long-short-term memory-based network and the generated confrontation network include a generator and a discriminator, the generator includes a first fully connected layer, multiple convolutional layers, and multiple atte...
Embodiment 2
[0106] On the basis of embodiment 1, this embodiment proposes a kind of motor imagery classification system based on CNN classification model and generation confrontation network, including:
[0107] The preprocessing module is used to eliminate part of the noise through ICA for the collected MI raw data, eliminate unimportant or interfering signals through a band-pass filter, and perform feature extraction through wavelet transform and fast Fourier transform;
[0108] The data expansion module is used to generate high-quality MI data based on long-term short-term memory network and generative confrontation network;
[0109] Data classification is used to improve classification performance and obtain classification results through multi-output convolutional neural networks.
[0110] Further, the data expansion module includes:
[0111] A generator unit for generating realistic MI data and establishing a mapping relationship between categories and data;
[0112] The filter un...
Embodiment 3
[0115] Such as Figure 4 , on the basis of embodiment 1, this embodiment proposes a terminal device based on CNN classification model and generative confrontation network motor imagery classification, terminal device 200 includes at least one memory 210, at least one processor 220 and a system connected to different platforms bus 230 .
[0116] Memory 210 may include readable media in the form of volatile memory, such as random access memory (RAM) 211 and / or cache memory 212 , and may further include read only memory (ROM) 213 .
[0117] Wherein, the memory 210 also stores a computer program, and the computer program can be executed by the processor 220, so that the processor 220 executes any one of the above-mentioned motor imagery classification methods based on the CNN classification model and the generation confrontation network in the embodiment of the present application, wherein The specific implementation manner is consistent with the implementation manners and achiev...
PUM
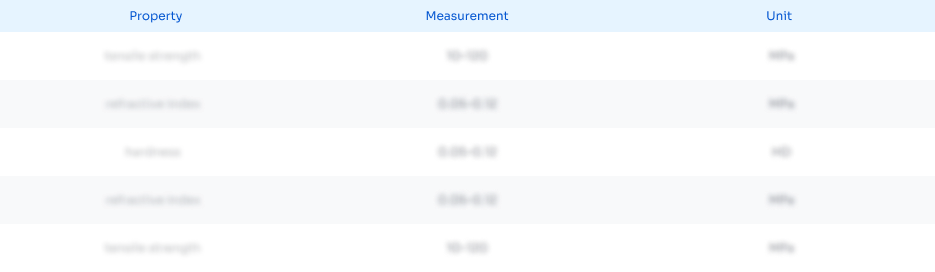
Abstract
Description
Claims
Application Information
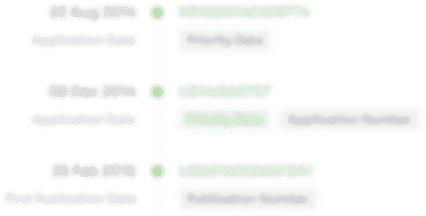
- R&D Engineer
- R&D Manager
- IP Professional
- Industry Leading Data Capabilities
- Powerful AI technology
- Patent DNA Extraction
Browse by: Latest US Patents, China's latest patents, Technical Efficacy Thesaurus, Application Domain, Technology Topic, Popular Technical Reports.
© 2024 PatSnap. All rights reserved.Legal|Privacy policy|Modern Slavery Act Transparency Statement|Sitemap|About US| Contact US: help@patsnap.com