Accelerated execution method of deep learning model in dynamic change network environment
A network environment and deep learning technology, applied in the field of edge computing and the Internet of Things, can solve the problems of inability to model segmentation, large time complexity, and availability impact, and achieve the effect of accurate segmentation and speeding up the data analysis process.
- Summary
- Abstract
- Description
- Claims
- Application Information
AI Technical Summary
Problems solved by technology
Method used
Image
Examples
Embodiment Construction
[0042] Below in conjunction with specific embodiment, further illustrate the present invention, should be understood that these embodiments are only used to illustrate the present invention and are not intended to limit the scope of the present invention, after having read the present invention, those skilled in the art will understand various equivalent forms of the present invention All modifications fall within the scope defined by the appended claims of the present application.
[0043] A method for accelerating the execution of a deep learning model in a dynamically changing network environment, including 1) obtaining the actual running time of each layer of the convolutional neural network on the edge and the cloud and the output size of each layer; monitoring the network bandwidth in real time, according to the neural network The output size of each layer and the network bandwidth get the transmission delay. 2) The layers of the neural network are abstracted into nodes,...
PUM
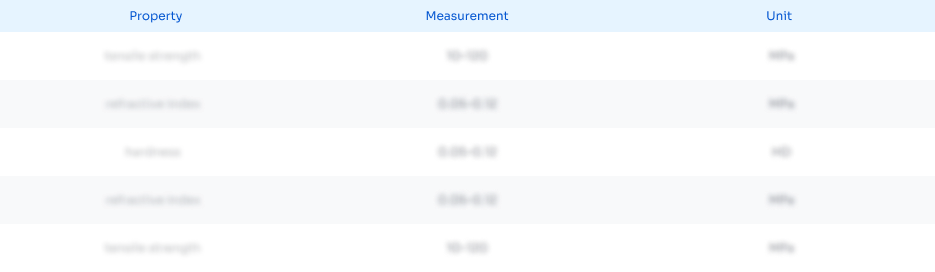
Abstract
Description
Claims
Application Information
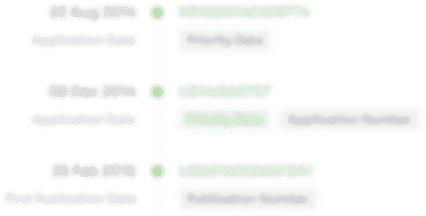
- R&D
- Intellectual Property
- Life Sciences
- Materials
- Tech Scout
- Unparalleled Data Quality
- Higher Quality Content
- 60% Fewer Hallucinations
Browse by: Latest US Patents, China's latest patents, Technical Efficacy Thesaurus, Application Domain, Technology Topic, Popular Technical Reports.
© 2025 PatSnap. All rights reserved.Legal|Privacy policy|Modern Slavery Act Transparency Statement|Sitemap|About US| Contact US: help@patsnap.com