Water quality BOD measurement method based on AQPSO-RBF neural network
A neural network and measurement method technology, applied in the design field of water quality BOD multi-source sensors, can solve the problems of complex operation process, difficult maintenance of equipment, long detection time, etc., and achieve the effect of short time consumption, reduced manpower and convenient operation.
- Summary
- Abstract
- Description
- Claims
- Application Information
AI Technical Summary
Problems solved by technology
Method used
Image
Examples
Embodiment Construction
[0074] The present invention will be further described below in conjunction with the accompanying drawings.
[0075] refer to Figure 1 to Figure 5 , a kind of water quality BOD measuring method based on AQPSO-RBF neural network, comprises the following steps:
[0076] Step 1: Data preprocessing, the process is as follows:
[0077] The invention selects BOD in water quality pollution as a research target, and implements high-precision prediction of BOD through a technical solution of water quality BOD multi-source sensor based on AQPSO-RBF. The data set used in the water quality BOD model of AQPSO-RBF includes sensor data of temperature, dissolved oxygen, pH, oxidation-reduction potential, chemical oxygen demand, and BOD.
[0078] Step 1.1: Use the Raida criterion for data cleaning, delete outliers, and set the entire data set as x1, x2,...,xn. Using ei to represent the residual error of the data, the formula for the standard deviation is:
[0079]
[0080] If the resid...
PUM
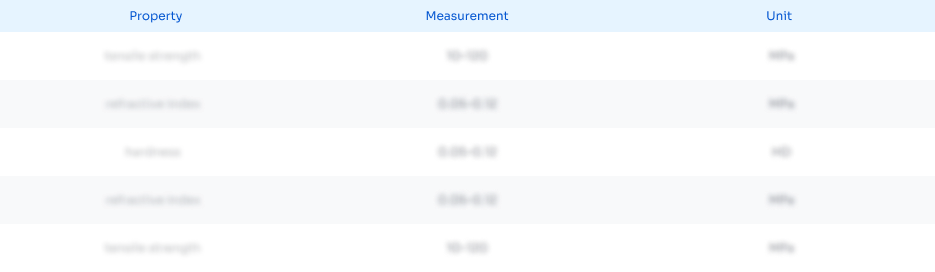
Abstract
Description
Claims
Application Information
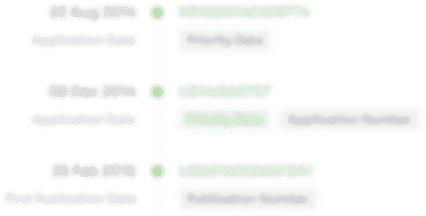
- R&D Engineer
- R&D Manager
- IP Professional
- Industry Leading Data Capabilities
- Powerful AI technology
- Patent DNA Extraction
Browse by: Latest US Patents, China's latest patents, Technical Efficacy Thesaurus, Application Domain, Technology Topic, Popular Technical Reports.
© 2024 PatSnap. All rights reserved.Legal|Privacy policy|Modern Slavery Act Transparency Statement|Sitemap|About US| Contact US: help@patsnap.com