Training method of personalized model of distillation-based semi-supervised federated learning
A training method and semi-supervised technology, applied in the field of personalized model training, can solve the problems of different degrees of importance, uneven data quality, not aggregation methods, etc., to reduce weights, improve performance, and achieve good performance.
- Summary
- Abstract
- Description
- Claims
- Application Information
AI Technical Summary
Problems solved by technology
Method used
Image
Examples
Embodiment Construction
[0023] In order to make the object, technical solution and advantages of the present invention clearer, the present invention will be further described in detail below in conjunction with specific examples.
[0024] We define k ∈ K clients owning a local dataset D k , where D k Include labeled local datasets and the unlabeled local dataset Local data for each client k and tend to different distributions, and N u >>N l . In order for the client models to observe on the same dataset, we share the same unlabeled shared data on each client N p >>N l .
[0025] Taking the medical scene as an example, the clients participating in the federated learning training are hospitals in different regions, and the local data sets are medical imaging data sets, such as neuroimaging data of Alzheimer's disease. The label of the data indicates whether it is sick or not.
[0026] see figure 1 , a training method for a personalized model based on distillation-based semi-supervised...
PUM
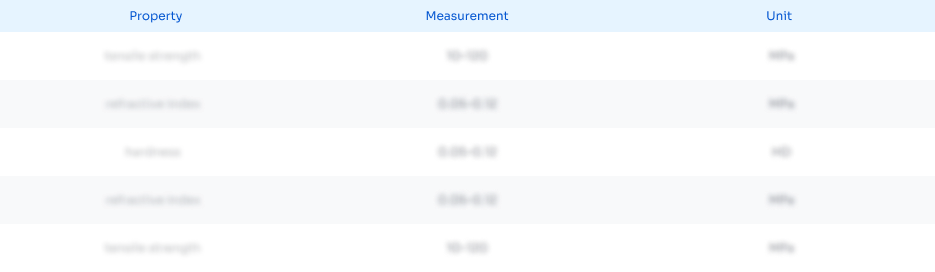
Abstract
Description
Claims
Application Information
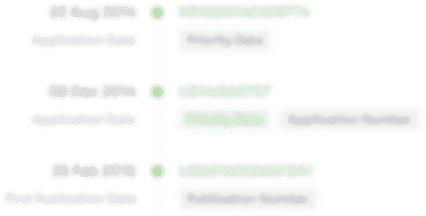
- R&D
- Intellectual Property
- Life Sciences
- Materials
- Tech Scout
- Unparalleled Data Quality
- Higher Quality Content
- 60% Fewer Hallucinations
Browse by: Latest US Patents, China's latest patents, Technical Efficacy Thesaurus, Application Domain, Technology Topic, Popular Technical Reports.
© 2025 PatSnap. All rights reserved.Legal|Privacy policy|Modern Slavery Act Transparency Statement|Sitemap|About US| Contact US: help@patsnap.com