Space-time image texture direction detection method and system based on DCNN and transfer learning
An image texture and direction detection technology, applied in neural learning methods, image data processing, image analysis and other directions, can solve problems such as low signal-to-noise ratio, inability to eliminate image noise, false detection, etc., to eliminate image noise and improve detection accuracy. rate effect
- Summary
- Abstract
- Description
- Claims
- Application Information
AI Technical Summary
Problems solved by technology
Method used
Image
Examples
Embodiment 1
[0027] like Figure 1 ~ 6 As shown, a time-space image texture direction detection method based on DCNN and migration learning, including: a time and space image; a predictive time-space image texture direction prediction model based on deep convolutional neural network and migration learning, Get the prediction result in the temporal image texture direction.
[0028] like Figure 5 As shown, a two-layer convolutional layer base layer, four convolution layer groups (first to fourth volume group), all-connection layer, and all-in-plane texture, and four convolutional layer groups (first to fourth volume layers), full connecting layer and Return layer. Specifically, including 2 layer convolution layer base layer, 9 layers of convolution layer constitutes a first rolling group, and the 12-layer convolution layer constitutes a second roll group, and the 69-layer convolution layer constitutes a third volume group. The 9-layer convolution layer constitutes a fourth volume group; each of t...
Embodiment 2
[0056] According to an embodiment, a time-space image texture direction detecting method based on DCNN and migration, this embodiment provides a time and space image texture direction detection system based on DCNN and migration learning, including: data acquisition module for collecting time and space images Time and Space Image Texture Direction Prediction Module, used to introduce the acquisition time and time-space image input well prediction model based on a deep convolutional neural network and migration learning, and acquire prediction results in the temporal image texture direction.
PUM
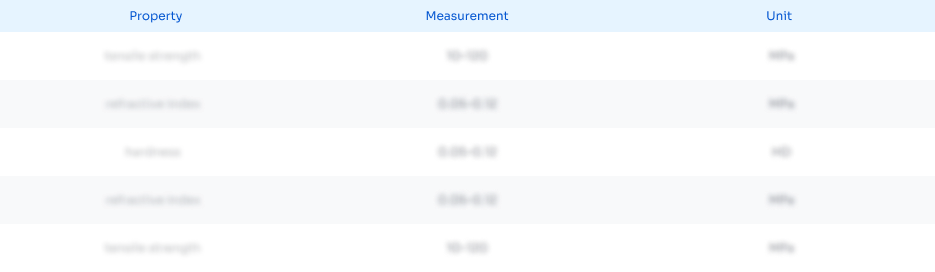
Abstract
Description
Claims
Application Information
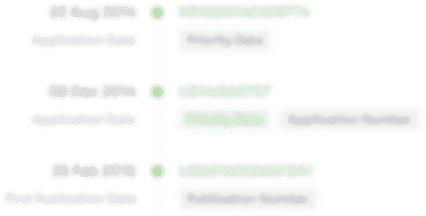
- Generate Ideas
- Intellectual Property
- Life Sciences
- Materials
- Tech Scout
- Unparalleled Data Quality
- Higher Quality Content
- 60% Fewer Hallucinations
Browse by: Latest US Patents, China's latest patents, Technical Efficacy Thesaurus, Application Domain, Technology Topic, Popular Technical Reports.
© 2025 PatSnap. All rights reserved.Legal|Privacy policy|Modern Slavery Act Transparency Statement|Sitemap|About US| Contact US: help@patsnap.com