Deep learning-based severe convection extrapolation method and system under multiple scales
A deep learning and strong convection technology, applied in the field of strong convection extrapolation methods and systems, can solve the problems of rapid deterioration in the quality of the extrapolation effect and poor MSE performance, and achieve the effect of improving performance and generalization ability
- Summary
- Abstract
- Description
- Claims
- Application Information
AI Technical Summary
Problems solved by technology
Method used
Image
Examples
Embodiment 1
[0078] refer to figure 1 It is a structural diagram of a multi-scale strong convective extrapolation system based on deep learning in the present invention. Specifically, the system includes:
[0079] Feature extraction module 1 is used to receive radar image data and extract hidden state features of radar image data;
[0080] In this embodiment, the feature extraction module 1 includes a VGG16 network, and the VGG16 network is used to extract the Doppler radar base data of the radar image data, and then extract the hidden state features of the radar base data according to the Doppler radar base data; wherein,
[0081] Doppler radar base data includes radar basic reflectivity, radar combined reflectivity, radar basic radial velocity, and combined radial velocity.
[0082] Further, the VGG16 network adopts convolutional layers by stacking small-sized convolutional kernels.
[0083] The strong convective extrapolation module 2 is connected with the feature extraction module, a...
Embodiment 2
[0098] refer to figure 2 and image 3 , which is a flowchart of a multi-scale strong convective extrapolation method based on deep learning in this embodiment, image 3 In BN, normalization is to normalize each batch of data, and tanh is a hyperbolic tangent function. The method includes the following steps:
[0099] S1: Receive radar image data and extract hidden state features of radar image data;
[0100] In this step, the Doppler radar base data of the radar image data is extracted through the VGG16 network, such as the radar basic reflectivity at an elevation angle of 0.5°, the radar combined reflectivity, the radar basic radial velocity at an elevation angle of 0.5°, and the combined radial velocity Wait;
[0101] refer to Figure 4 It is the structure diagram of the VGG16 network model in this embodiment. Among them, the first one is the original diagram. The VGG16 network in this embodiment is different from the usual ones. The input Doppler radar base data is imp...
PUM
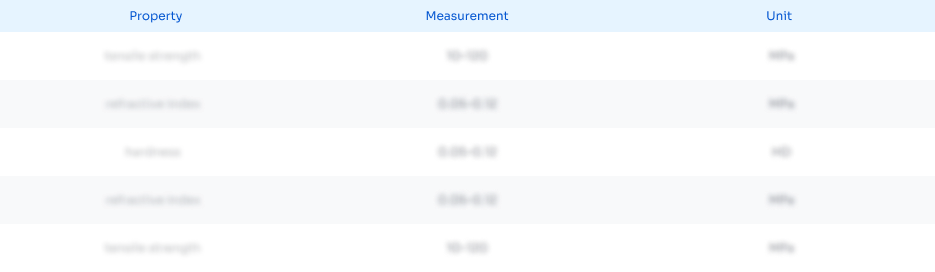
Abstract
Description
Claims
Application Information
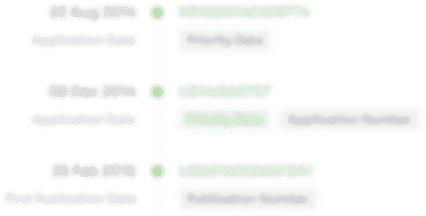
- R&D Engineer
- R&D Manager
- IP Professional
- Industry Leading Data Capabilities
- Powerful AI technology
- Patent DNA Extraction
Browse by: Latest US Patents, China's latest patents, Technical Efficacy Thesaurus, Application Domain, Technology Topic, Popular Technical Reports.
© 2024 PatSnap. All rights reserved.Legal|Privacy policy|Modern Slavery Act Transparency Statement|Sitemap|About US| Contact US: help@patsnap.com